Artificial intelligence, aka AI, has been the latest buzz, and it continues to make strides. Companies are continuously eyeing the potential of using AI in their operations, and they can’t have enough. From healthcare to the IT sector, these technological advancements are gaining immense traction.
This brings us to the topic of artificial intelligence (AI) in networking. According to a recent survey, 54% of organizations recognized cost savings and efficiencies as the primary advantages of AI adoption. Let’s learn more about it.
What is Artificial Intelligence (AI) in Networking?
AI for networking is a subset of AIOps (artificial intelligence for IT operations) focused on applying AI to network performance and operations.
Networking systems are getting more complex with digital transformation, multi-cloud, more devices and data, hybrid work, and more sophisticated attacks. As network complexity grows and changes, organizations need network operators to evolve too. Right talent shortages and limitations on budget only add to the problem. To solve this problem, organizations are turning to AI for networking.
How AI Is Used In Networking?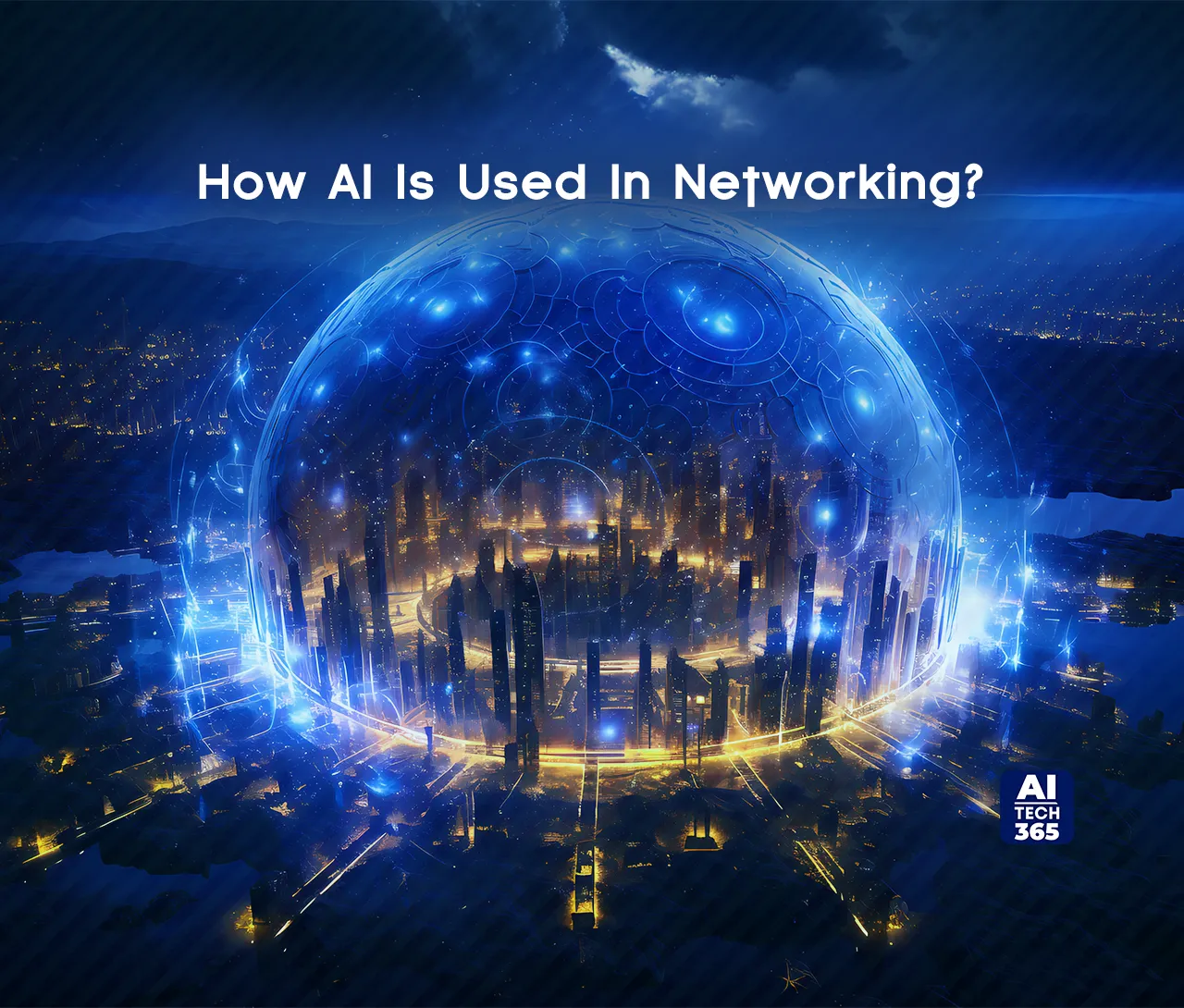
AI is used in networking to help with troubleshooting, issue resolution, and remediation guidance and to give you critical insights to improve the user and application experience. It enhances security by improving threat response and mitigation, customizes network analytics for accurate issue detection, and reduces unknowns in decision-making.
AI simplifies network management, automates tasks, and detects anomalies based on established norms for proactive response. However, there are implementation challenges, resistance to change, compatibility issues, and dependencies on other factors.
Advantages of AI in Networking
AI has many benefits in networking.
- Better Performance: AI can see patterns in the network data and fix issues before they happen.
- Security: AI can detect and respond to threats in real-time, provide threat intelligence, and automate security.
- Network Management: AI does network management tasks like configuration, monitoring, and troubleshooting, which reduces manual work and improves ops efficiency.
- Predictive Analytics: AI can see into historical network data, do proactive maintenance, and minimize downtime.
- Intelligent Automation: AI does the routine network tasks so IT can focus on strategic stuff.
- Faster Troubleshooting: AI tools can look at tons of network data, find the root cause, and give you the answer to fix it.
- Capacity Planning: AI can analyze traffic patterns and predict future capacity requirements, helping organizations optimize network resources and plan for growth.
Artificial Intelligence (AI) in Networking Use Cases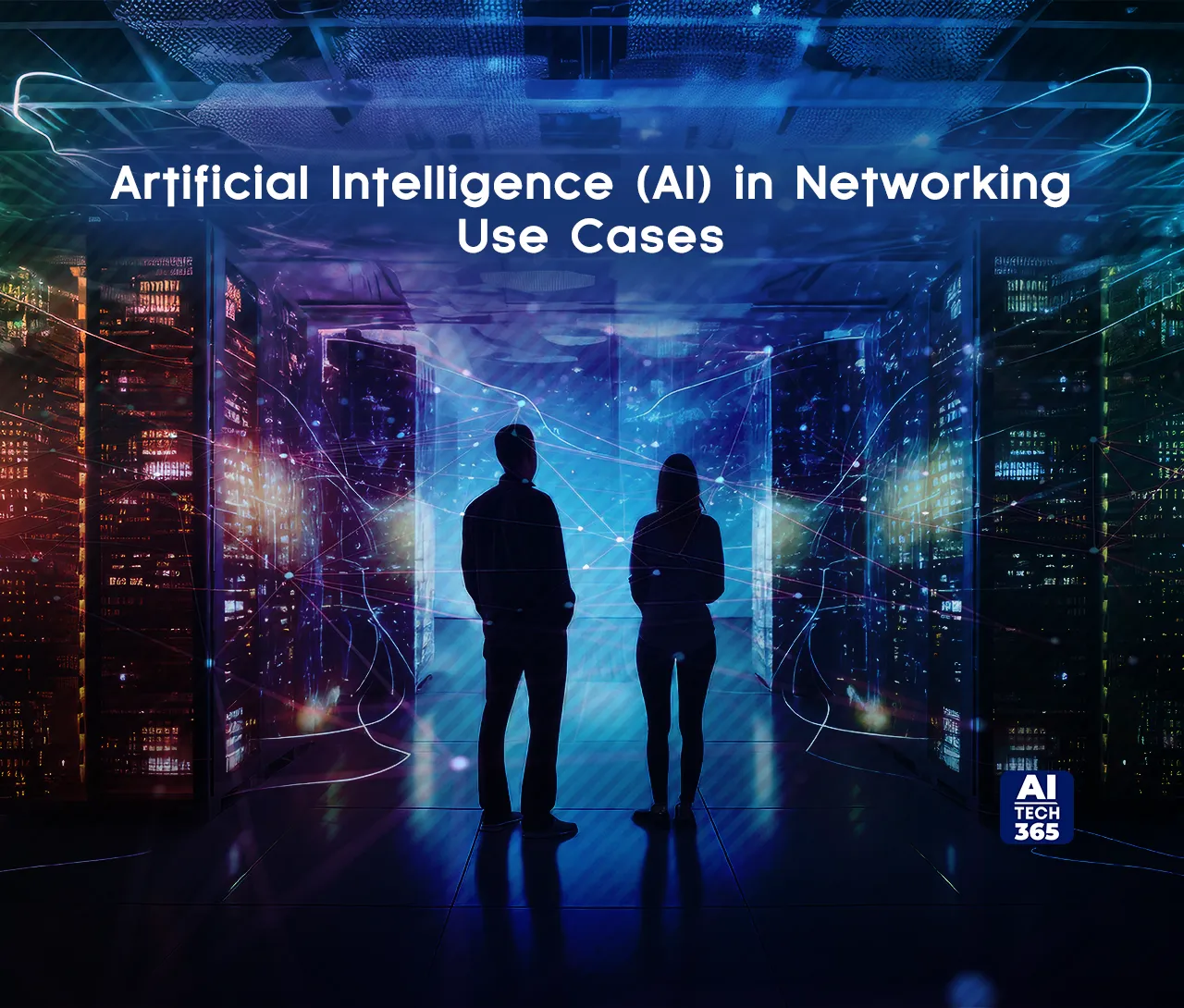
AI/ML for Wi-Fi Optimization
With machine learning, NetOps can be alerted to issues with network congestion, Wi-Fi interference, and office traffic loads. System-generated insights can forecast future events and notify IT to take corrective action by figuring out how a sequence of events is related to one another.
Machine Learning For Policy Automation
Through the analysis of endpoint groups’ traffic flows, machine learning can yield information about source and destination, service, protocol, and port numbers. Policies that permit or prohibit interactions between various groupings of devices, people, and apps can be defined using these traffic insights.
Machine Reasoning For Lifecycle Management
Machine reasoning can go through thousands of network devices to check that all devices have the latest software image and look for configuration vulnerabilities. If an ops team is not using the latest upgrade features, it can flag suggestions.
What To Search For In A Networking Solution Using AI?
IT teams are unable to meet the needs of today’s networks without an appropriate AI strategy. Observe the following:
- The correct data: An enormous amount of high-quality data is the foundation of every significant AI networking system. AI learns over time by gathering and analyzing data. The AI system gets smarter the more diverse the data it collects. Furthermore, domain-specific data labeling aids in the training of AI models. For instance, the network user experience can be categorized and tracked using design intent metrics, which are categories of structured data.
- The appropriate answer: An effective artificial intelligence (AI) for networking solutions should offer real-time insights, minimize alert fatigue by giving concerns priority, and provide suggested repair activities. An AI engine processes such data using a variety of AI approaches, generally referred to as a data science toolbox, to produce the appropriate response. Neural networks and other supervised and unsupervised ML and DL techniques should be used to evaluate network data and produce useful insights.
- The appropriate infrastructure: To gather, process, and deliver an intelligent answer, a strong and scalable infrastructure is needed. A dependable and adaptable infrastructure for data collection and processing that can grow to accommodate AI workloads both today and in the future is offered by cloud-hosted processing.
Also Read: 10 Examples of AI as a Service (AIaaS) You Need to Know
What Are The Challenges of AI in Networking
Did you know that big data, cloud computing, and artificial intelligence (AI) are among the most popular technologies that organizations will probably use; by 2027, 75% of enterprises expect to have implemented them. That’s incredible!
However, before AI in networking becomes mainstream, it has a few hurdles to overcome, such as:
- Complexity and integration: Nowadays, networks are getting more complex with various interconnected components, data sources, and interfaces. To incorporate AI solutions, we need to rethink our existing networks and make them capable of handling this complexity.
- Data integrity and security: AI heavily relies on processing vast amounts of high-quality data. Any new AI networking solutions must prioritize data integrity, security, and privacy from the get-go. We can’t compromise on that!
- Interoperability and open standards: Dealing with data from different network vendors can be quite a challenge. Each vendor may have its own data format and syntax, making it difficult to work with. Thankfully, open standards like Open RAN are evolving and should help tackle this issue in the future.
- Skills gap and retraining: Integrating AI in networking means that network engineers will have to adapt to a new way of working. As AI technologies advance, they’ll need to learn new skills and methods to deploy and maintain AI-powered networks. It’s a shift in the working culture, but it’s well worth it!
So, as much as we’d love to see a world of automated, software-defined, self-defending networks right away, we still have some ways to go. But hey, progress takes time, right?
End Note
AI in networking is a game changer. With enterprise AI tools, you can boost network performance, security, and management, and make better decisions.
With AI tools, you get proactive troubleshooting, predictive analytics, and clever automation. That means better efficiency, less downtime, and a great user experience. As AI keeps evolving, it’s got the potential to totally shake up how networks are managed and operated, giving organizations the power to stay ahead in our crazy connected digital world.