Deep learning is one of the effective approaches to train computers to execute tasks that come naturally to humans. Deep learning algorithms have various applications across different sectors. For example, these models are utilized for developing driverless cars. Deep learning algorithms use neural decoding to identify a stop sign or determine the difference between a pedestrian and a lamp post.
This blog discusses the intersection of deep learning and neuroscience. It focuses on how artificial neural networks (ANNs) are being applied to study the brain, particularly in the field of social neuroscience.
What is Deep Learning in Neuroscience?
The field of systems neuroscience delves into understanding how the brain accomplishes a wide range of tasks, from sensory perception to cognitive processing and movement. On the other hand, artificial intelligence focuses on developing computational systems depending on the tasks they will have to execute. The following are the three key elements in artificial neural networks (ANNs):
- The objective function
- Rules established for learning
- The architecture
Deep learning continues to thrive, leveraging brain-inspired architectures. These above-mentioned three core components have become crucial to how we design, build, and refine complex artificial learning systems.
Benefits of Deep Learning in Neuroscience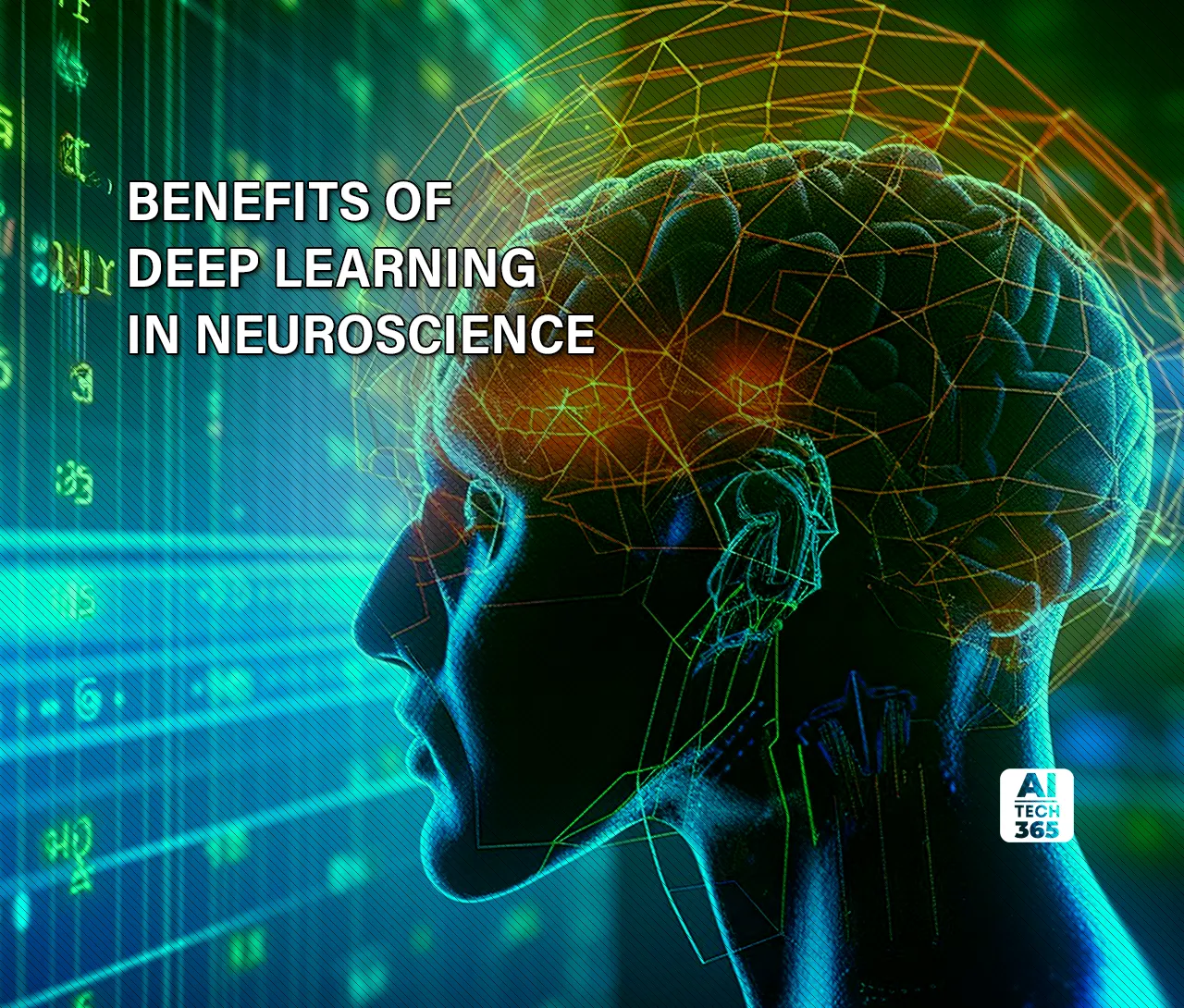
Here are a few advantages of using deep learning in neuroscience:
1. Automated Feature Development
Deep learning algorithms can automatically create new features from a limited set found in the training data. It eliminates the need for manual intervention. This allows deep learning to tackle complex tasks that typically demand significant feature engineering. For businesses, this translates to quicker deployment of applications or technologies with improved accuracy.
2. Seamlessly Works With Unstructured Data
One of the most significant advantages of deep learning is its capacity to process unstructured data. This capability is important for businesses, as most of their data is unstructured. Whether it’s text, images, or voice, these data formats are prevalent across industries. Traditional machine learning algorithms struggle to effectively analyze unstructured data, leaving much of its value untapped. This is where deep learning steps in, offering transformative potential. By training deep learning models on unstructured data with proper labeling, businesses can enhance a wide range of functions, from marketing and sales to finance.
3. Efficient Self-Learning Abilities
Various layers in deep learning networks enable models to be more effective at learning complex features and executing intricate computational tasks. For instance, it has the capability to perform complex operations simultaneously. Deep learning performs exceptionally well compared to machine learning in tasks related to machine perception, including unstructured datasets. This is possible because of deep learning models’ capability to learn from their own mistakes. These models can validate the correctness of its forecasts/results to make necessary adjustments. Traditional machine-learning models need various degrees of human intervention to understand the accuracy of the results. The performance of deep learning models is directly related to the volume of training data sets. As a result, if the data sets are bigger, organizations will have more accuracy.
4. Scalability
Deep learning models have better scalability because of their functionality to process huge amounts of data and execute multiple computations in a cost and time-efficient manner.
Deep learning is highly scalable due to its ability to process massive amounts of data and perform a lot of computations in a cost- and time-effective manner. This has a direct impact on productivity, enabling faster deployments and rollouts while also enhancing modularity and portability, as trained models can be applied to a variety of problems. For example, Google Cloud’s AI platform prediction allows businesses to run deep neural networks at scale on the cloud. Beyond better model organization and version control, companies can take advantage of Google’s cloud infrastructure to efficiently scale batch predictions. This improves overall efficiency by automatically adjusting the number of nodes in use based on the volume of requests.
Also Read: Top 6 Benefits of Integrating AI Servers
Use Cases of Deep Learning in Neuroscience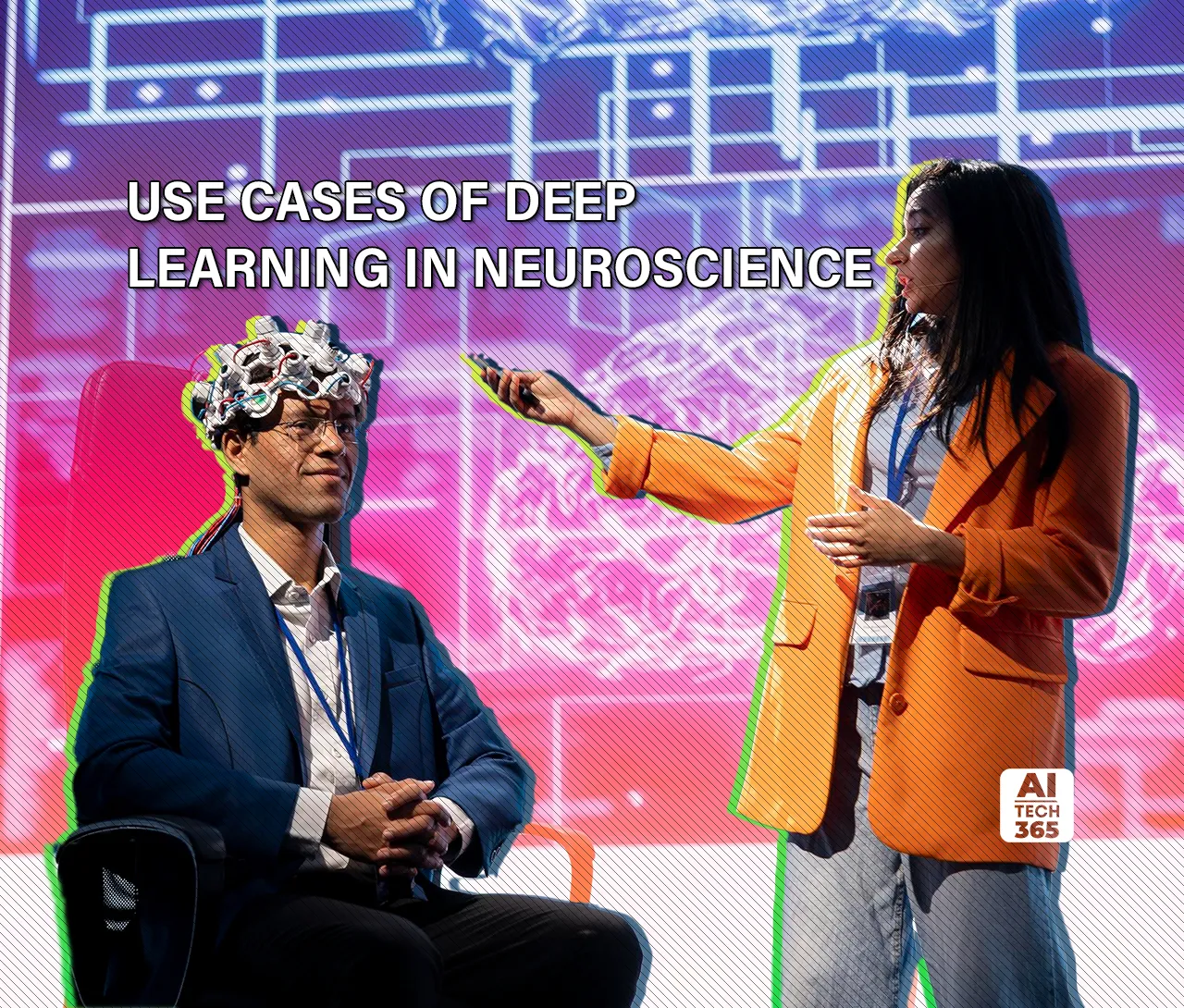
Here are a few applications of deep learning in neuroscience:
1. Building Predictive Models
Deep learning models, such as convolutional neural networks (CNNs), are being applied to predict mental health conditions using brain imaging data. These models can identify non-linear relationships between brain activity and behavior, improving over traditional linear models.
2. Modeling Brain Functions
ANNs, particularly CNNs, are used to model various brain functions. For instance, CNNs have been shown to model human visual behavior and its corresponding neural representations effectively, offering some of the highest fits to fMRI data in the visual cortex.
3. Generating and Annotating Data
ANNs are applied in neuroscience to automatically annotate and manipulate naturalistic stimuli, such as social interactions, making it easier to process complex stimuli in large datasets. They can also be used to generate new data, such as artificial brain data for training models.
4. Cognitive and Neural Modeling
Deep learning models can be used to simulate brain-like processes, allowing researchers to create computational models of cognition that mimic human brain functions and behaviors. This includes modeling human biases, interactions, and brain coupling during social exchanges.
Challenges and Ethical Concerns With Deep Learning In Neuroscience
While ANNs offer significant promise, they require large datasets and are prone to biases that can affect results. There are also concerns about interpretability, as neural networks may learn misleading “shortcuts” that don’t reflect true neural mechanisms.
Wrapping it Up
This blog emphasizes that deep learning could revolutionize neuroscience by helping model brain imaging, neural decoding, and behavior more precisely. It also warns of the complexity, data demands, and ethical challenges involved.