Machine learning is not a new term. Especially with AI tools being developed for almost every other field, this aspect of computing is widespread. But can we say the same about quantum machine learning (ML)?
The short answer is no! Quantum machine learning is a relatively new field, and it is still in the development process. In layman’s terms, it is like giving super-smart quantum computers the ability to learn new things fast. As of now, there are no real-life applications out for public use.
However, IBM is heavily invested in such research processes and you can even set up an account for exploring the same. Drug discovery, financial modeling, material science, etc are the use cases of this approach. In this article, we have explained how it is different from classical approaches.
What Is Quantum Machine Learning?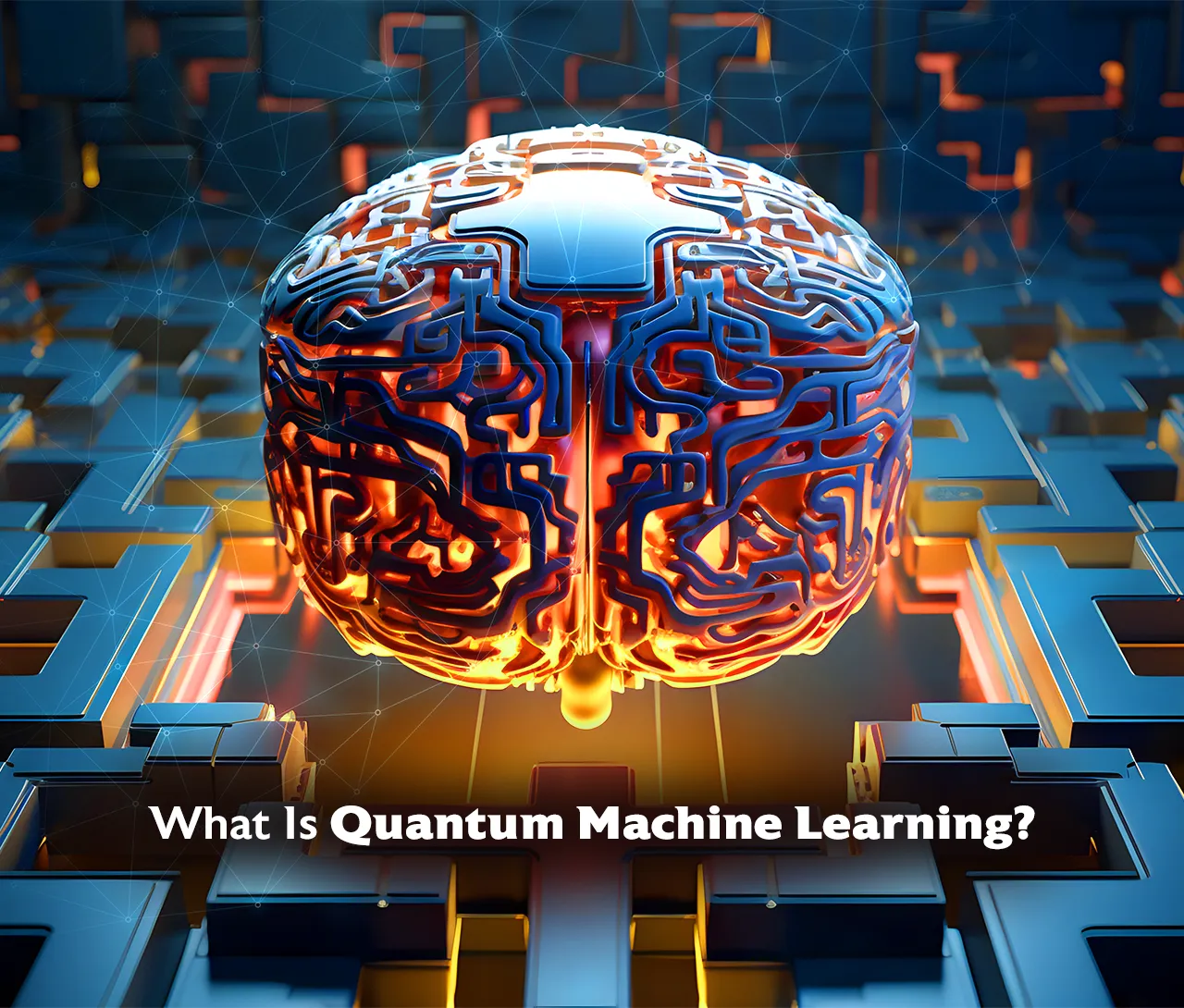
The study of the nexus between quantum computing and machine learning is known as quantum machine learning. It’s about advancing machine learning methods and algorithms by utilizing the ideas and powers of quantum computing.
Quantum ML consists of two fundamental components:
- Quantum-Enhanced Machine Learning: The employment of quantum algorithms to solve machine learning issues more correctly or effectively than conventional algorithms is known as “quantum-enhanced machine learning.” Certain calculations and optimizations can be accelerated using quantum algorithms by taking advantage of quantum features like superposition and entanglement.
Quantum algorithms, such as the Variational Quantum Eigensolver (VQE) and the Quantum Approximate Optimization Algorithm (QAOA), can be used to improve reinforcement learning or optimize control parameters. - Quantum Machine Learning: In quantum ML, data from quantum systems or experiments is analyzed and comprehended by the application of classical machine learning algorithms. This covers things like designing new quantum experiments or understanding the phase transitions of a quantum system.
Researchers can learn more about quantum systems and improve their comprehension of quantum events by applying classical machine-learning techniques.
Types of Quantum Machine Learning
Quantum Neural Networks (QNN)
These are artificial neural networks that are powered by qubits, the fundamental building blocks of quantum information, and quantum logic gates. Quantum phenomena like superposition and entanglement, in which qubits can exist in numerous states simultaneously and be correlated with one another, can be used by QNNs. QNNs are more effective and quicker learners than traditional neural networks.
Quantum Reinforcement Learning (QRL)
These are algorithms for reinforcement learning that learn the best policies and actions by utilizing quantum settings and quantum agents. Quantum resources are utilizable by QRL.
Quantum Support Vector Machines (QSVM)
These are vector-based machine learning models that determine the ideal hyperplane to divide the data points into distinct classes using quantum methods. Quantum speedup and quantum parallelism, which allow quantum computers to perform several tasks concurrently and solve complicated problems more quickly than classical computers, are capabilities that QSVMs can leverage.
Also Read: What Is AI Hardware and What are its Key Components?
Uses of Quantum Machine Learning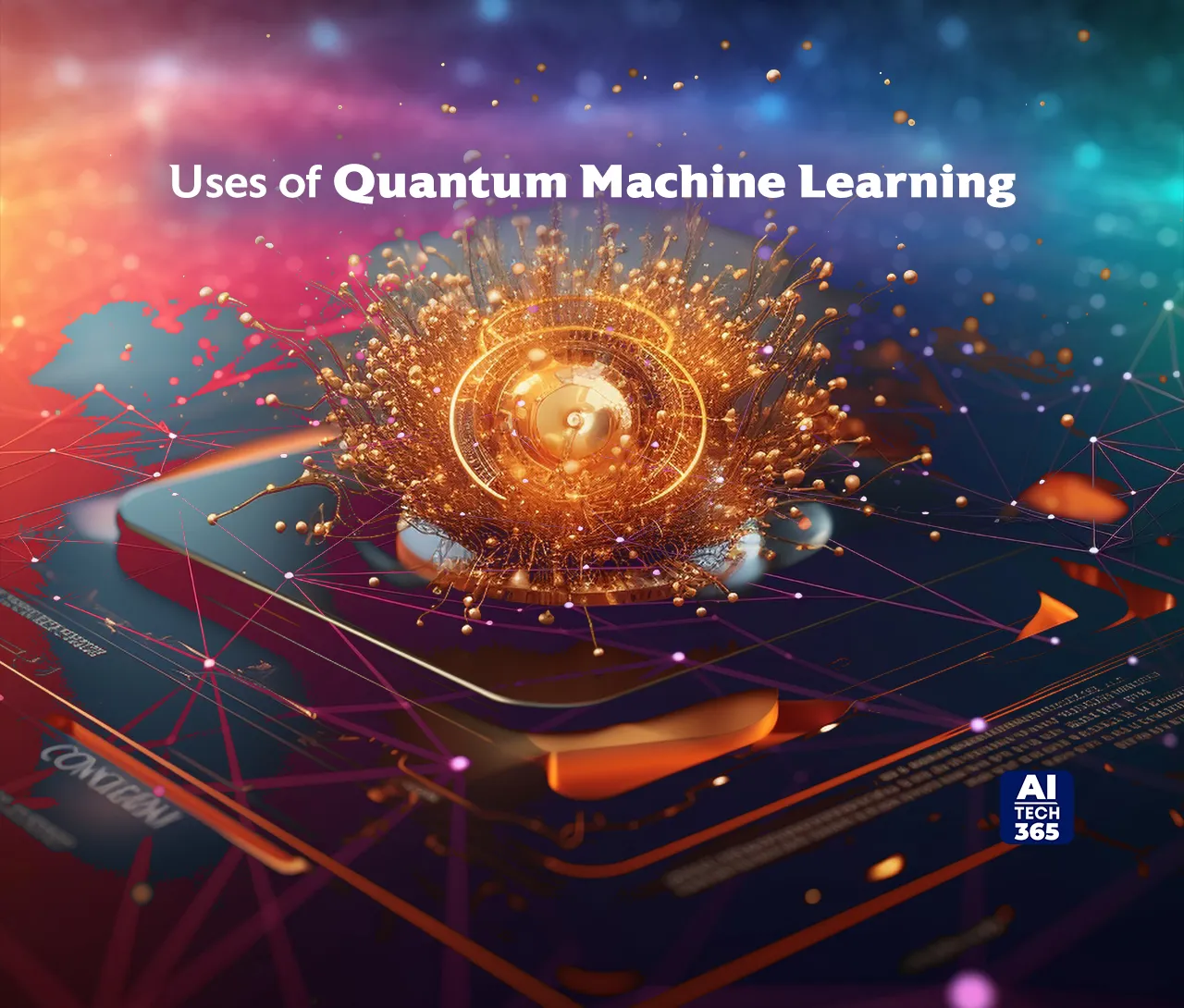
Quantum ML has many use cases across many domains. Here are some:
- Pattern Recognition: Pattern recognition tasks such as speech and picture identification can be enhanced by quantum ML. Large datasets can be processed and analyzed more quickly and precisely using quantum algorithms.
- Drug Discovery: It can be used to speed up the search for novel pharmaceuticals. Chemical structures and attributes can be analyzed using quantum ML algorithms to find and maximize potential medications.
- Quantum Chemistry: Complex quantum systems in chemistry can be simulated and understood using quantum ML. In addition to simulating chemical reactions and optimizing quantum circuits for quantum chemistry simulations, it can forecast the characteristics of molecules.
- Financial Modeling: Financial modeling and forecasting can be done with quantum ML techniques. Large financial datasets can be analyzed by them to identify trends, forecast stock market trends, evaluate risk, and optimize portfolios.
- Natural Language Processing: Text summarization, sentiment analysis, and language translation are examples of jobs that quantum ML can enhance. Language data may be processed and analyzed more quickly and precisely by quantum algorithms.
- Data analysis: Big, complicated datasets can be analyzed using machine learning quantum to uncover hidden patterns and glean insightful information. Data visualization, anomaly detection, and grouping are among its applications.
- Quantum Generative Models: Based on discovered patterns, generative models that produce fresh data samples can be developed using quantum. This can be used to create artificial data for quantum system simulation and machine learning model training.
Quantum Machine Learning vs Quantum Computing
The study of creating and utilizing quantum systems for calculations, utilizing their features to solve some problems tenfold quicker than with traditional computers, is known as quantum computing. However, quantum ML improves machine learning procedures and algorithms by utilizing the concepts of quantum computing. It investigates how quantum devices and algorithms can enhance machine learning tasks’ data analysis, optimization, and processing speed. Quantum ML especially exploits these ideas to improve machine learning skills, whereas quantum computing concentrates on the creation of quantum systems.
Winding Up
The combination of machine learning with quantum computing is represented by quantum machine learning. Through the utilization of quantum qualities and techniques, quantum ML holds the capability to transform numerous fields. It offers improved optimization, more complex data processing, and more effective computations. Quantum ML, however still in its early stages, holds great potential to address difficult problems and expand our understanding of both quantum systems and machine learning methods. As long as this field of study and development is pursued, we can look forward to groundbreaking discoveries and innovative applications.