Cybercrime is expected to cost the world $10.5 trillion every year by 2025. This shows that we really need to improve cybersecurity to protect against these huge losses. More and more companies need to use machine learning in cybersecurity to protect themselves from malicious attacks. These attacks keep getting more common and complicated, but machine learning is getting better at finding them early. It can analyze lots of data to spot patterns, which helps it find weaknesses in networks and predict when and how future attacks might happen.
Let’s jump in and understand everything about machine learning in cybersecurity.
What is Machine Learning?
Machine learning (ML) is a part of artificial intelligence (AI) that teaches computers to learn from data and make decisions without specific instructions. It works by training algorithms on past data to recognize patterns and connections, allowing them to predict outcomes or take actions with new information.
How Machine Learning Is Used In Cybersecurity?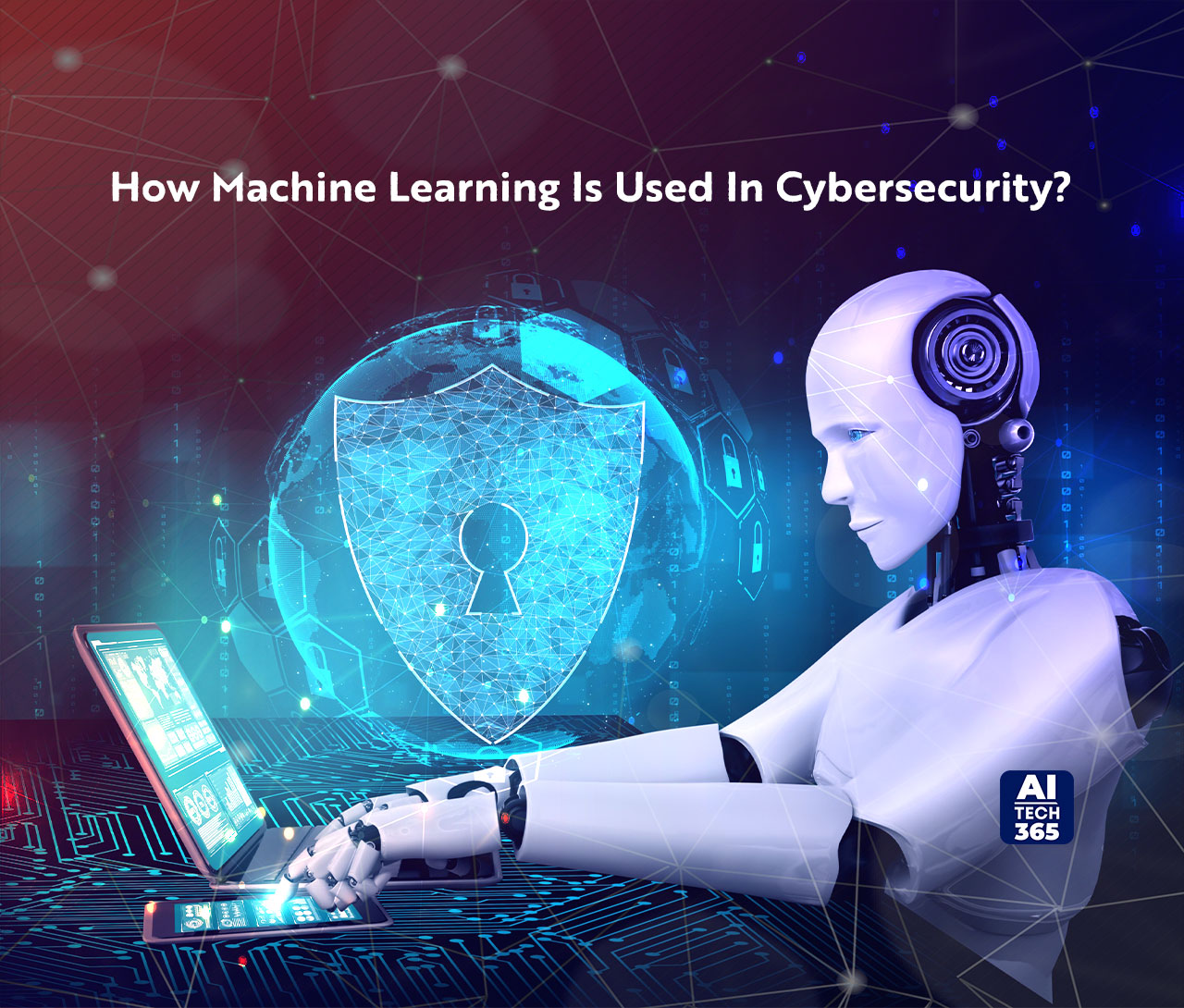
AI and machine learning in cybersecurity is used in multiple ways, including:
Detecting Threats in Early Stages
Machine learning is increasingly playing a critical role in cybersecurity by detecting threats in their early stages. With its capacity to sift through vast amounts of data, machine learning algorithms can identify potentially harmful files, allowing organizations to intervene before these threats escalate. A notable example is Microsoft’s Windows Defender, which utilized machine learning to thwart a large-scale cyber attack in 2018, preventing over 400,000 users from falling victim to a cryptocurrency mining scheme within a mere 12-hour window.
Uncovering Network Vulnerabilities
ML enables companies to adopt a proactive approach to cybersecurity by uncovering network vulnerabilities before malicious actors exploit them. Through processes like penetration testing, machine learning algorithms simulate cyber attacks to pinpoint weaknesses in a company’s networks, firewalls, and systems. These algorithms can then apply necessary software patches and fixes, bolstering an organization’s overall security posture. Additionally, by analyzing historical data, machine learning algorithms can detect unusual software and user behavior during training sessions, helping organizations prioritize and mitigate potential threats based on their specific vulnerabilities.
Reducing IT Workloads and Costs
ML’s automation capabilities offer significant benefits in reducing IT workloads and costs. Tasks such as distributing security updates, conducting penetration tests, and monitoring devices can be automated, freeing up IT teams to focus on more critical security issues. This automation not only improves efficiency but also enables organizations to save on hiring costs, as machine learning can fulfill roles that would otherwise require additional personnel. Particularly for smaller businesses with limited resources, leveraging machine learning in cybersecurity provides a cost-effective solution to enhance their security posture without increasing headcount.
Benefits of Machine Learning in Cybersecurity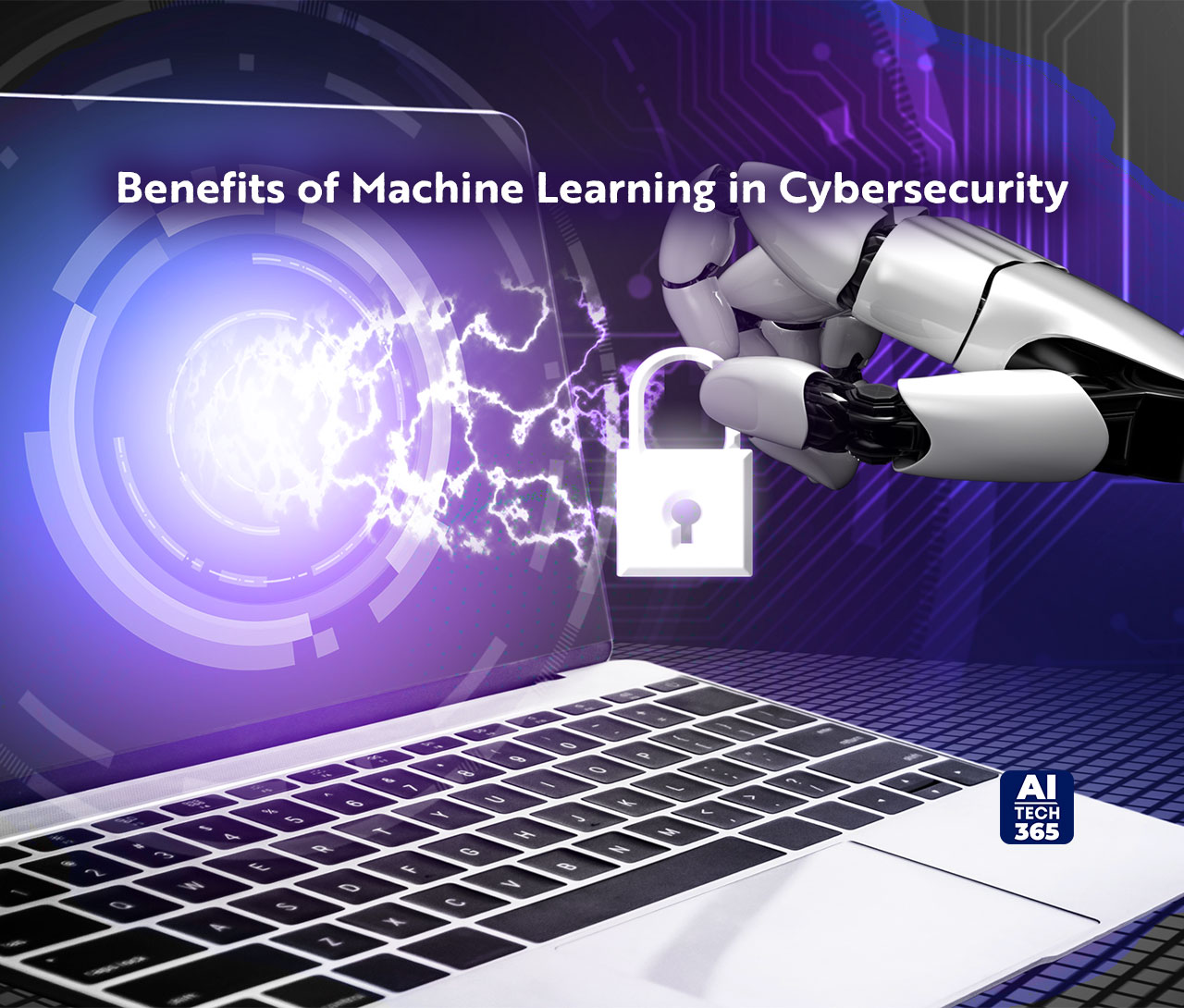
Below are the numerous benefits offered by machine learning in cybersecurity.
- Automated Cybersecurity Processes: Machine learning can learn new functions and improve existing ones autonomously, leading to automated workflows. This liberates security and IT teams from mundane tasks, enabling them to concentrate on tackling emerging cyber threats, resolving critical vulnerabilities, and accomplishing more sophisticated assignments.
- Ability to Handle Large Data Sets: Machine learning excels at processing and analyzing large volumes of data swiftly. Algorithms can identify trends faster than humans, alerting teams to emerging cyber attacks. This enables IT and security personnel to take immediate action, stopping cyber attacks in their tracks before they escalate.
- Protected Security: By scrutinizing a company’s security infrastructure, machine learning algorithms can pinpoint weaknesses, suggest remedies, and assist teams in preparing for various cyber attacks. This proactive approach enables security and IT teams to preemptively address threats, establishing robust procedures and systems to thwart complex attacks.
- Adaptable Defense Systems: Machine learning not only prepares for known cyber threats but also anticipates future attacks that may be unfamiliar to organizations. Security teams can enhance their companies’ resilience by fortifying their security technology stacks and educating employees about new social engineering schemes and other cyber attacks.
- Minimized Human Errors: Unlike human employees, machine learning offers comprehensive protection round-the-clock without fatigue. Additionally, it can learn from its experiences and insights to continually enhance its performance. This allows security teams to delegate more operations to highly trained algorithms, reducing human errors that could otherwise occur.
Examples of Machine Learning in Cybersecurity
- Anomaly Detection: Machine learning algorithms can detect anomalies in network traffic patterns, indicating potential cyber threats such as unauthorized access or malware activity. By analyzing historical data and learning normal behavior, these algorithms can identify deviations from the norm, alerting security teams to investigate and mitigate potential threats.
- Phishing Detection: Machine learning models can analyze email content and sender behavior to identify phishing attempts. By training on large datasets of known phishing emails and legitimate correspondence, these models can learn to recognize common phishing tactics, such as spoofed email addresses or suspicious links, and flag suspicious messages for further review by security analysts.
- Endpoint Security: Machine learning is used in endpoint security solutions to detect and prevent malware infections on individual devices. By continuously analyzing file behavior and system activities, machine learning algorithms can identify malware signatures and behavioral patterns, enabling real-time threat detection and response to protect endpoints from malicious attacks.
Future of Machine Learning in Cybersecurity
The rise of remote work and hybrid work models has increased the need for machine learning in cybersecurity. As systems generate vast amounts of data, machine learning’s ability to quickly scan and analyze this data is critical. However, cyber threats are adapting, making it difficult to detect harmful data or code. To address this, the cybersecurity industry is developing new roles and best practices, such as ethical hacking, deception technology, and analyzing human behavior to detect cyber risks.