In the high-stakes arena of cybersecurity, the rules of engagement are shifting. Threat actors are getting smarter. They use automation and artificial intelligence for constant attacks. Traditional defense mechanisms can’t keep up. Generative Artificial Intelligence (GenAI) is changing how organizations see and tackle cyber threats. It helps them anticipate, neutralize, and outsmart risks in new ways. AI tech leaders must grasp GenAI’s role in cybersecurity. It’s not just a smart move; it’s essential for survival.
The Limitations of Traditional Cybersecurity
For decades, cybersecurity has operated on a reactive model. Organizations used signature-based detection and firewalls. They also relied on manual threat-hunting teams to find known malware and suspicious patterns. But this approach has glaring flaws. Signature-based tools are good for known threats. However, they have trouble with new attack methods. Hackers take advantage of this delay. They use zero-day exploits and polymorphic malware that changes to avoid being detected.
Traditional cybersecurity measures often lag in detecting breaches. For instance, in 2021, the average time to identify and contain a data breach was 287 days—212 days to detect and 75 days to contain.
The sheer volume of data further complicates defense. Security teams get flooded with alerts. Many are false positives. This causes alert fatigue and leads to missed threats. It still takes months to spot a breach, industry reports say. This gives attackers plenty of time to steal data or insert ongoing threats.
GenAI’s Predictive Power Starts From Anomaly Detection to Proactive Defense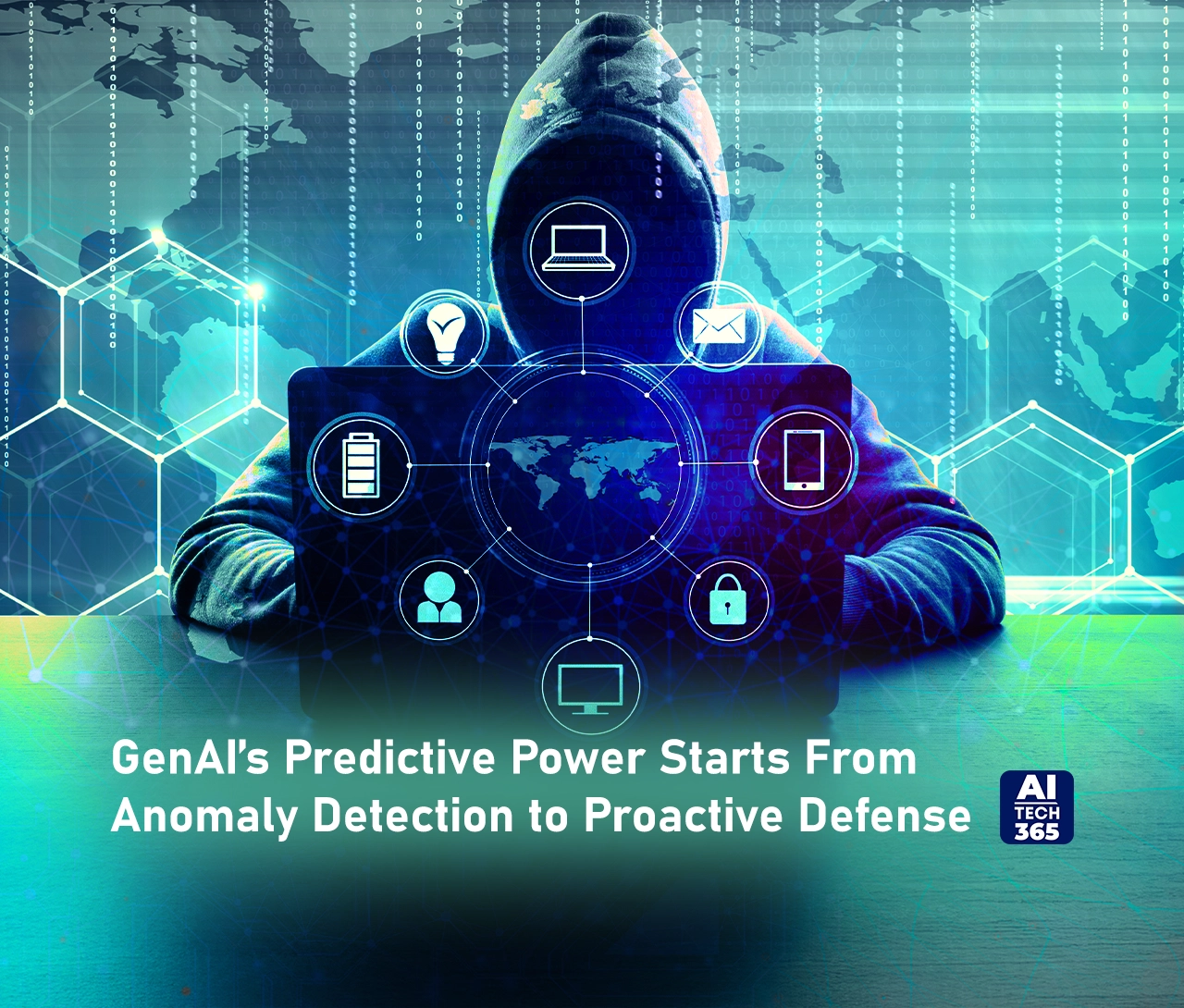
GenAI flips the script by enabling predictive cybersecurity. GenAI models are different from traditional tools. They don’t wait for an attack to happen. Instead, they look at past data, network behavior, and global threats. This helps them predict attacks before they occur. These systems are great at spotting small anomalies. This could be a small shift in user activity, a sudden jump in data transfers, or a weird login from a new place.
Take, for example, behavioral analytics. GenAI models use large datasets to spot patterns for each user, device, and app in an organization. If a finance employee opens sensitive files at midnight, the system flags it for review. GenAI provides detailed visibility not just for people. It also watches machine identities, APIs, and IoT devices. This way, no endpoint escapes scrutiny.
Proactive threat hunting is another game-changer. By simulating potential attack scenarios, GenAI uncovers vulnerabilities that human analysts might overlook. Think of a model that creates fake phishing emails. It tests how well employees resist them and checks for weak spots in network defenses. These simulations harden systems and give useful insights. This helps improve incident response protocols.
Real-Time Prevention is Automating Defense at Machine Speed
Prediction is only half the battle. The true value of GenAI lies in its ability to act instantaneously. When a threat is found, automated systems kick in. They isolate affected devices, revoke access, or launch countermeasures; all without needing humans. This speed is critical. A delay of even a few minutes can turn a small incident into a major breach. The effectiveness of AI in cybersecurity is evident. MIT’s AI2 system, for example, demonstrated the capability to detect 85% of attacks, significantly outperforming previous benchmarks.
Adaptive defense mechanisms further enhance resilience. GenAI doesn’t just follow pre-programmed rules; it evolves. These systems improve their strategies by looking at past incidents. They learn to spot new attack signatures and tactics used by adversaries. If a hacker breaks up malicious code to avoid detection, the AI adjusts its scanning methods. This helps it find the scattered payloads.
Case Studies Where GenAI is in Action
The real-world impact of GenAI is already evident. A global financial institution recently stopped a ransomware attack. They used an AI-based network monitoring tool to do it. The system found unusual encryption activity on multiple servers. It quickly isolated the affected systems. Then, it stopped the threat before any data was stolen. The financial sector remains a prime target, with breaches averaging a cost of US$ 5.72 million in 2021.
Another example comes from a healthcare provider leveraging GenAI for endpoint security. The AI found several unauthorized access attempts. They were disguised as regular user logins. Further analysis revealed a coordinated brute-force attack targeting patient records. The organization blocked the IP addresses and used multi-factor authentication. This helped prevent a possible HIPAA violation. Even nation-state actors are feeling the heat. Cybersecurity companies use GenAI to study malware linked to advanced persistent threats (APTs). These tools analyze code and link it to geopolitical events. This helps spot state-sponsored campaigns early, so defenses can be set up in advance.
Ethical Considerations and Challenges
While GenAI’s potential is immense, its adoption isn’t without risks. Privacy concerns top the list. To train well, GenAI needs a lot of sensitive data. This includes employee behaviors, customer information, and network logs. Organizations must balance security and ethical data practices. They need to comply with regulations like GDPR and CCPA.
Adversarial attacks pose another challenge. Hackers are now targeting AI systems. They feed these systems poisoned data to change their outcomes. A good adversarial attack can mislead GenAI. It might classify malware as safe or miss a key vulnerability. To reduce this risk, we need strong model checks. We also require constant monitoring and “red team” drills to test our defenses.
Human oversight remains indispensable. While GenAI excels at pattern recognition and automation, it lacks contextual understanding. A sudden rise in data traffic could mean an attack or a real marketing campaign. Final decisions should be made by skilled analysts. This is important, especially when there are legal or reputational risks involved.
The Future of GenAI in Cybersecurity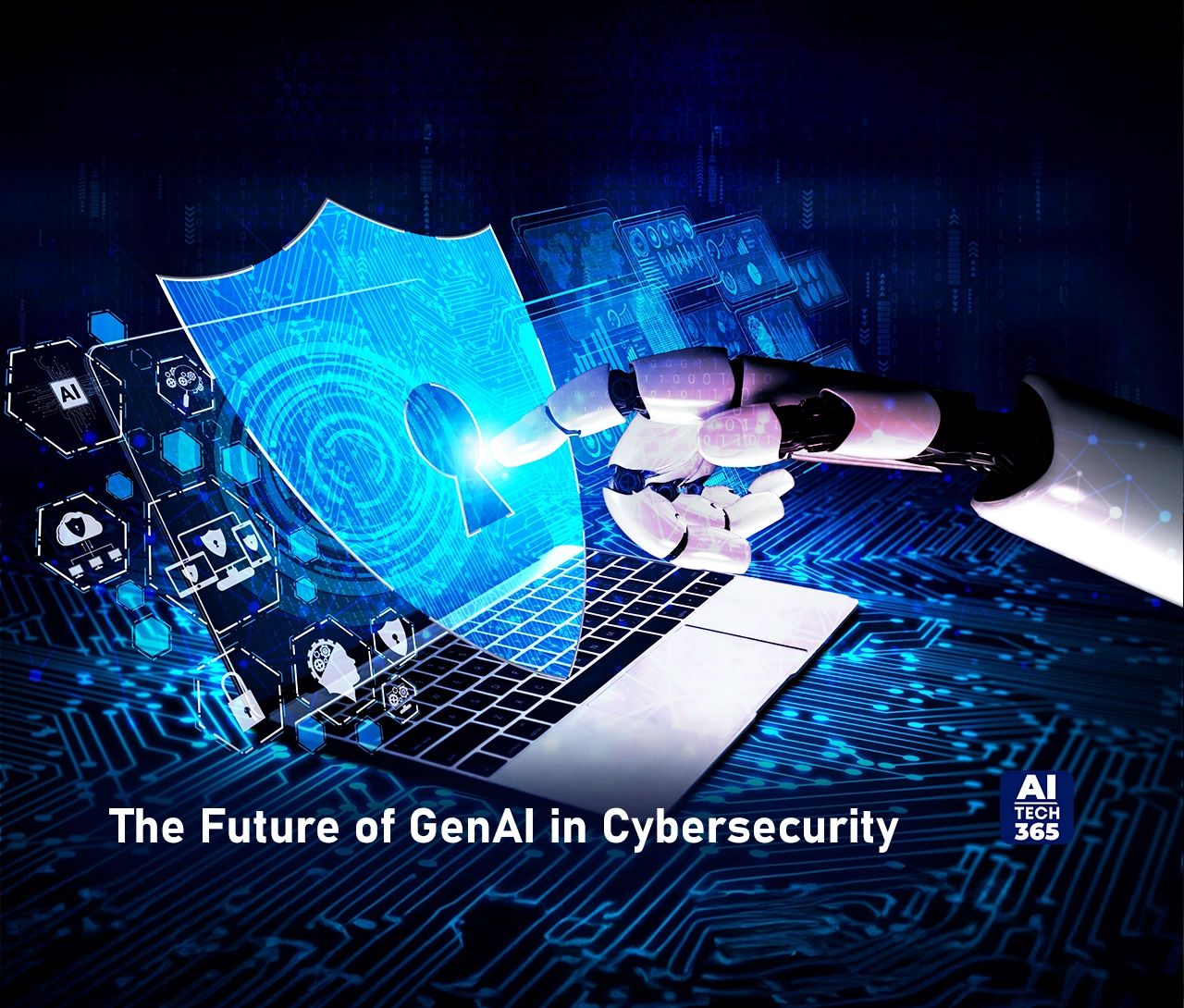
Looking ahead, GenAI will become the backbone of self-learning security ecosystems. Picture networks that share threat intelligence on their own across different industries. This would form a united defense against cyber threats. It’s like a collective immune system. A telecom company breach can lead to swift updates for healthcare providers, banks, and government agencies. This helps set up barriers before attackers can move.
Collaboration between humans and AI will also deepen. GenAI can analyze unstructured data like emails, social media, and dark web forums. This helps analysts find hidden threats. An AI scanning dark web chats can spot talks about a new ransomware attack. This helps organizations fix weaknesses before they face threats.
Democratization is another frontier. Small and mid-sized businesses often lack top-notch security resources. Now, they can access GenAI tools through cloud platforms. This levels the playing field, ensuring that even resource-constrained organizations can defend against sophisticated threats.
A Call to Action for Leaders
For leaders steering the future of AI and cybersecurity, complacency isn’t an option. Begin by reviewing the current setup. Look for gaps where GenAI can improve detection or response. Work with vendors that provide explainable AI solutions. These models give clear insights into how they make decisions. Upskill your teams. This will close the gap between cybersecurity skills and AI knowledge.
Finally, foster a culture of collaboration.
- Share anonymized threat data with industry peers.
- Contribute to open-source AI projects.
- Join cybersecurity consortiums.
Fighting cybercrime takes teamwork. GenAI can reach its full potential when we innovate together.
In the arms race between defenders and attackers, GenAI represents a paradigm shift. It predicts threats accurately and automates responses quickly. This offers a lifeline in a more unstable digital world. For AI tech leaders, the message is clear. The future of cybersecurity is not just about matching hackers. It’s about staying three steps ahead.