In the realm of artificial intelligence, where companies are increasingly integrating AI into their operations, the demand for transparency in decision-making processes has become paramount. As organizations embrace AI and sophisticated analytics for automating decisions, the necessity for understanding how these models arrive at conclusions grows more pronounced. This is where the significance of explainable AI techniques comes into play.
As we move forward in this blog, we will talk more about explainable AI methods. Let’s dig in!
What is Explainable AI?
Explainable AI, also known as XAI, encompasses a range of techniques and approaches that enable users to comprehend and have confidence in the outcomes generated by the machine learning algorithms of artificial intelligence systems. The accompanying explanations cater to different stakeholders, such as users, operators, and developers, and aim to tackle various concerns related to user acceptance, governance, and system development.
The ability to provide transparency and understandable justifications is essential for fostering trust and ensuring widespread adoption and benefits of AI in the market. Moreover, other correlated and emerging endeavors include initiatives focused on trustworthy and responsible AI.
What is the Algorithm for Explainable AI?
Explainable AI employs specific techniques to ensure traceability and understanding of each decision in the machine learning process. Unlike traditional AI, where the decision-making process might be opaque to its creators, XAI aims to enhance transparency and comprehensibility in AI-driven predictions.
By countering the “black box” nature of machine learning, XAI strives to improve users’ effectiveness by providing insights into the reasoning behind AI decisions. It aligns with the social right to explanation, enhancing user trust in AI systems. One notable algorithm within XAI adapts Shapley values, originally designed for fair payouts in cooperative games, to elucidate individual predictions in machine learning models.
How are Explainable AI Techniques Implemented?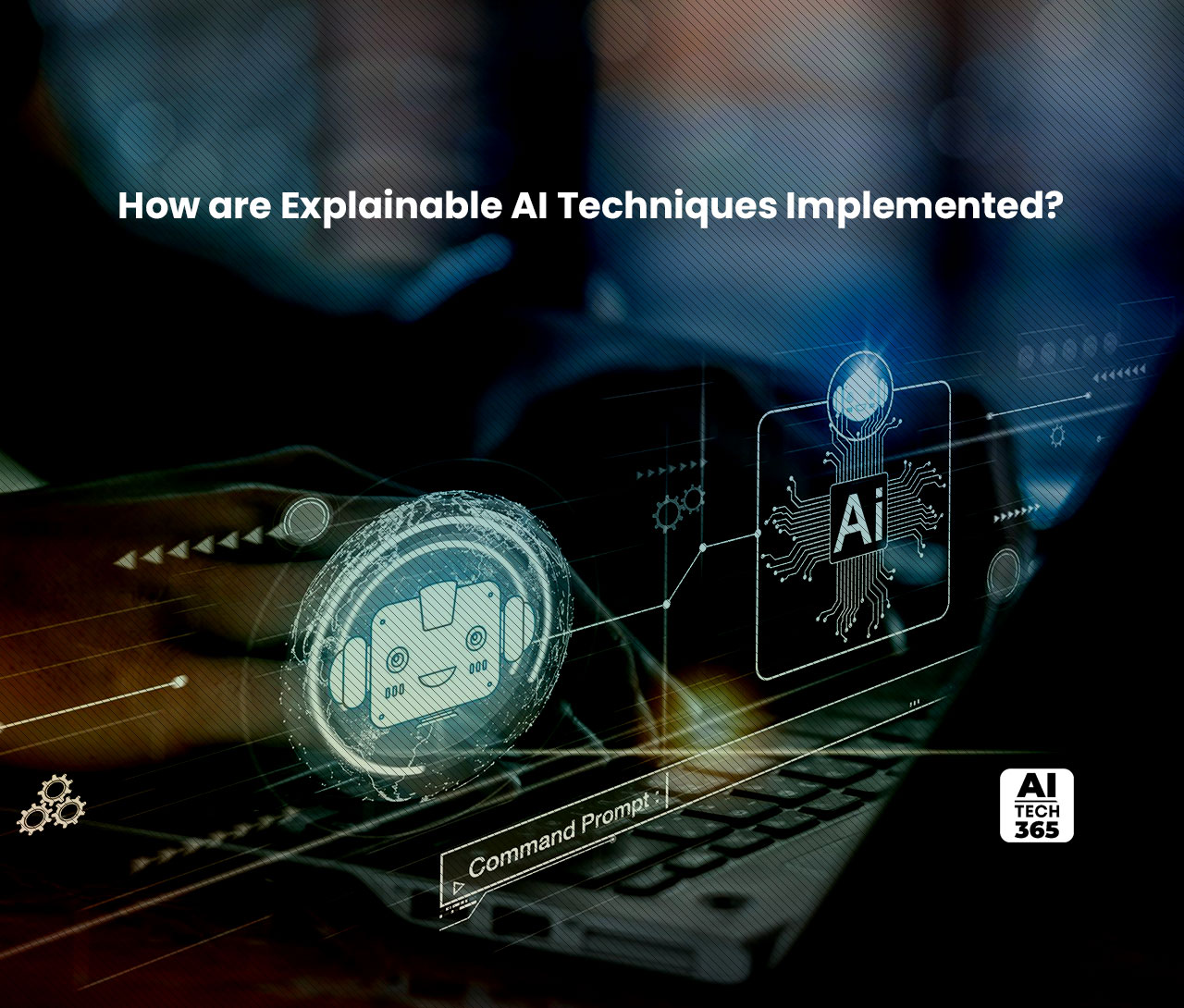
The U.S. National Institute of Standards and Technology (NIST) outlines four key principles guiding explainable AI:
- Explanation: Systems furnish supporting evidence or reasons for all outputs.
- Meaningful: Systems present explanations that are comprehensible to individual users.
- Explanation Accuracy: The provided explanation accurately reflects the system’s process for generating the output.
- Knowledge Limits: The system operates within predefined conditions or when output confidence levels are sufficient.
NIST emphasizes that the complexity of explanations may vary, catering to the needs of different consumers. The agency illustrates various explanation types under five non-exhaustive categories:
- User benefit
- Societal acceptance
- Regulatory and compliance
- System development
- Owner benefit
Explainable AI Techniques
The implementation of explainable AI methods encompasses three primary approaches. The technology requirements are covered by prediction accuracy and traceability, while the human aspect is addressed by decision understanding. The importance of explainable AI, particularly explainable machine learning, cannot be overstated as future warfighters must comprehend, trust, and proficiently handle a new wave of artificially intelligent machine collaborators.
Prediction accuracy
In order to ensure the effectiveness of AI in daily operations, accuracy plays a crucial role. To determine prediction accuracy, simulations are conducted and the output of XAI is compared to the results in the training dataset. Local Interpretable Model-Agnostic Explanations (LIME) is a widely used technique for explaining the predictions made by machine learning algorithms. It helps in understanding the prediction process of classifiers.
Traceability
Traceability is another one of the major explainable AI techniques. One way to accomplish this is by imposing restrictions on decision-making processes and establishing a more specific framework for machine learning rules and features. DeepLIFT, an XAI technique, exemplifies traceability by comparing the activation levels of individual neurons to their respective reference neurons, thus establishing a clear connection between activated neurons and revealing dependencies among them.
Decision understanding
The human factor plays a significant role in explainable AI techniques. Numerous individuals hold a sense of mistrust towards AI, however, to effectively collaborate with it, they must develop a level of trust. This can be achieved by providing proper education to the team that works with AI, enabling them to comprehend the rationale behind the AI’s decision-making process.
Best Practices to Implement Explainable AI Techniques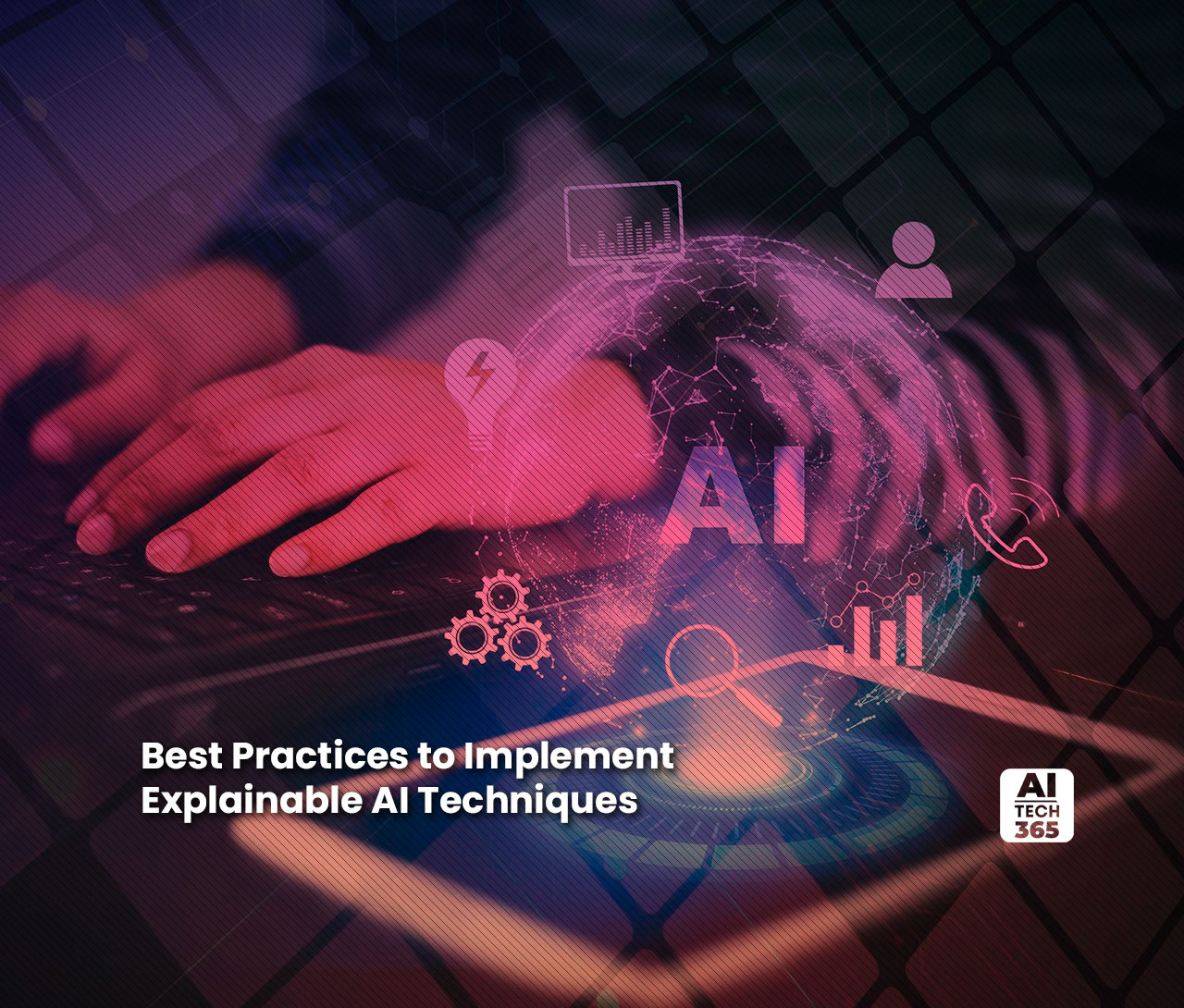
Here are key best practices for implementing Explainable AI (XAI) in your organization:
- Establish a diverse AI governance committee, comprising technical, business, legal, and risk experts. This committee guides AI development, defining organizational XAI frameworks, selecting appropriate tools, and setting standards based on use cases and risk levels.
- Invest in the right talent and tools for implementing XAI in your organization. Choose between custom, off-the-shelf, or open-source tools based on your short- and long-term requirements to stay current in this dynamic field.
- It is imperative to provide a clear and precise description of your specific use case or problem and the context in which your Explainable AI (XAI) will be utilized. This ensures that you have a thorough understanding of the distinct risks and legal obligations associated with each model.
- Tailor the explanation level of your XAI system to suit your audience’s understanding.
- Select the most suitable explainable AI techniques for the specific problem and scenario you have identified. This may involve considering techniques like feature importance analysis, model-agnostic approaches, or model-specific methodologies.
- To guarantee the accuracy and reliability of your XAI models, it is important to assess them using metrics like accuracy, transparency, and consistency. This evaluation process may involve considering the trade-offs between explainability and accuracy to make informed decisions.
- Validate your XAI models for any biases to ensure fairness and eliminate any forms of discrimination.
- It is crucial to consistently observe and revise your XAI models as necessary in order to uphold their precision, clarity, and impartiality.
As global governments regulate AI use, its explainability grows crucial. In the U.S., President Biden’s AI Bill of Rights and FTC’s (Federal Trade Commission) oversight underscore the importance of personal data protection. Explainable AI is key for addressing compliance, legal, and security risks in AI models.
To Sum it Up
Understanding the significance of explainable AI techniques is paramount in navigating the evolving landscape of artificial intelligence. By embracing transparency and comprehensibility in AI decision-making, organizations can enhance user trust, address legal and compliance concerns, and foster a more responsible AI ecosystem. As we delve into the future, the practical implementation of explainable AI techniques examples will undoubtedly play a pivotal role in shaping ethical and trustworthy AI systems.