In the high-stakes arena of modern enterprise technology, network failures are not just inconveniences—they’re existential threats. A five-minute outage for a Fortune 500 company can hemorrhage millions in revenue, erode customer trust, and trigger regulatory scrutiny. For instance, on July 19, 2024, CrowdStrike outage triggered a massive global IT disruption, affecting approximately 8.5 million Microsoft Windows systems worldwide.
As networks become more complex with hybrid clouds, IoT, and edge computing, traditional monitoring tools struggle to keep pace. Enter artificial intelligence: the champion, quietly rewriting the rules of network resilience.
For smart AITech leaders, AI-driven predictive network analytics is a must. It’s the new battlefield. This isn’t about following trends. It’s about survival. In today’s world, downtime means lost chances and damage to your reputation. Let’s dissect how AI is transforming network management from reactive firefighting to strategic foresight.
From Crystal Balls to Neural Networks: The Evolution of Failure Prevention
Predictive analytics isn’t unique. For decades, engineers relied on threshold-based alerts—setting rigid rules like “alert me when server CPU usage exceeds 90%.” But these binary systems lack nuance. They talk about symptoms while ignoring the condition. Modern AI, however, thrives on context. Machine learning models find early signs of failure by using historical data, real-time metrics, and outside factors like weather. These models can spot details that human analysts often overlook.
Consider how Nokia leverages AI to predict 5G network congestion. By analyzing traffic patterns, device types, and application demand, their systems automatically reroute data flows hours before users notice latency. The result? A 40% reduction in service degradation incidents across European telcos in 2023.
The Anatomy of AI-Powered Prediction: More Than Just Algorithms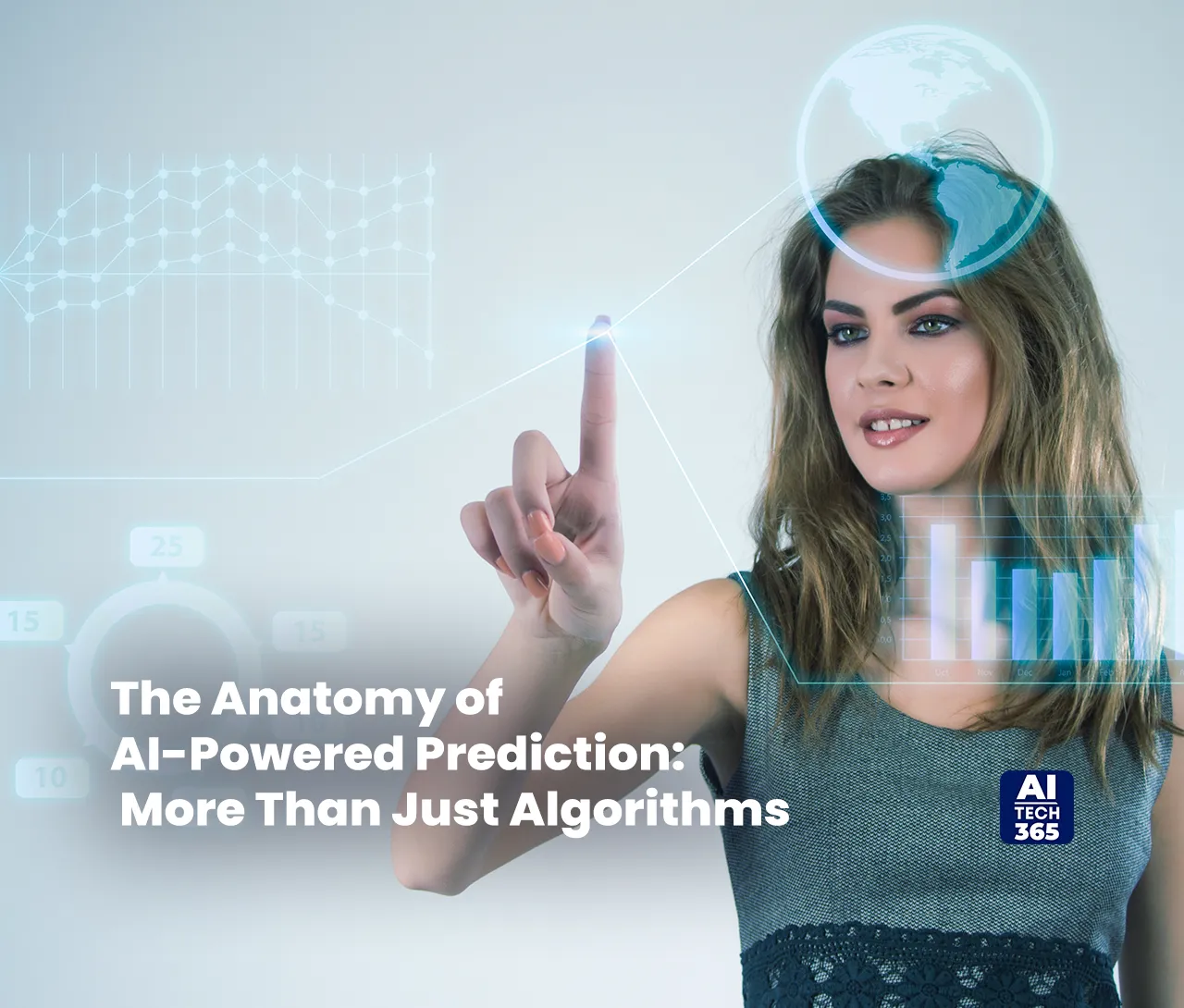
At its core, predictive network analytics relies on three AI pillars:
Data Synthesis Engine
Networks generate exabytes of logs, packet captures, and performance metrics daily. AI doesn’t just process this data—it curates it. Techniques like topological data analysis map relationships between network components, while federated learning enables models to train on distributed data without compromising privacy.
Temporal Pattern Recognition
Long short-term memory (LSTM) networks excel here. These neural architectures spot cyclical trends—like how database queries spike every fiscal quarter—and distinguish them from genuine anomalies. Palo Alto Networks’ Cortex XDR platform uses LSTMs to detect stealthy lateral movements in cyberattacks, cutting mean detection time from 48 hours to 19 minutes.
Probabilistic Failure Forecasting
Rather than binary “will fail/won’t fail” predictions, Bayesian networks assign probabilities. For instance, an AI might warn: “Router Cluster A has an 83% chance of packet loss exceeding SLAs within 72 hours due to firmware drift and rising ambient temperatures.” This granularity lets teams prioritize fixes strategically.
Real-World Impact: Case Studies in Preventative Intelligence
Case 1: The Cloud Provider That Outsmarted Latency
When a hyperscaler noticed intermittent latency in its East Asia regions, traditional tools blamed ‘network congestion.’ Their AI system dug deeper. By correlating submarine cable maintenance schedules, BGP routing changes, and even social media chatter about regional power outages, the model pinpointed an overlooked fiber route nearing capacity. Preemptive rerouting saved an estimated US$12 Mn in potential downtime costs during Lunar New Year traffic spikes.
Case 2: Manufacturing’s Predictive Pivot
A robotic assembly line for a German automaker began experiencing mysterious micro-outages. The culprit? AI cross-referenced motor vibration data with supplier logs to find a batch of subpar capacitors degrading faster than specs suggested. Replacement before failure avoided a 22-day production halt.
Also Read: How Applications of LLM Enhance Efficiency Across Multiple Fields
Navigating the Minefield: Challenges in AI-Driven Predictions
For all its promise, deploying AI in network analytics is not plug-and-play. Common pitfalls include:
- Data Myopia: Models trained only on internal data miss macro trends. A bank’s network AI failed to predict a DNS outage because it had not incorporated geopolitical risks affecting their third-party provider.
- Explainability Trade-offs: Deep learning models are notoriously opaque. When a major retailer’s AI recommended shutting down 30% of edge nodes preemptively, CTOs demanded a ”why. New techniques like SHAP (SHapley Additive exPlanations) are bridging this trust gap.
- Skill Chasms: 68% of enterprises in a 2024 Pluralsight survey admitted their network teams lack MLops expertise. Upskilling is non-negotiable.
The Horizon: Where AI Meets Autonomous Healing
The endgame is not prediction—it’s self-remediation. Imagine AI that doesn’t just flag a failing switch but negotiates with SDN controllers to isolate it, spins up virtual replacements, and dispatches a repair drone—all before humans get alerted. Early adopters like British Telecom are testing this with ‘network healing’ algorithms that resolve 31% of incidents autonomously.
Quantum machine learning looms as the next disruptor. By solving optimization problems intractable for classical computers, quantum-enhanced AI could dynamically redesign network topologies in real-time during crises.
Actionable Blueprint for AITech Leaders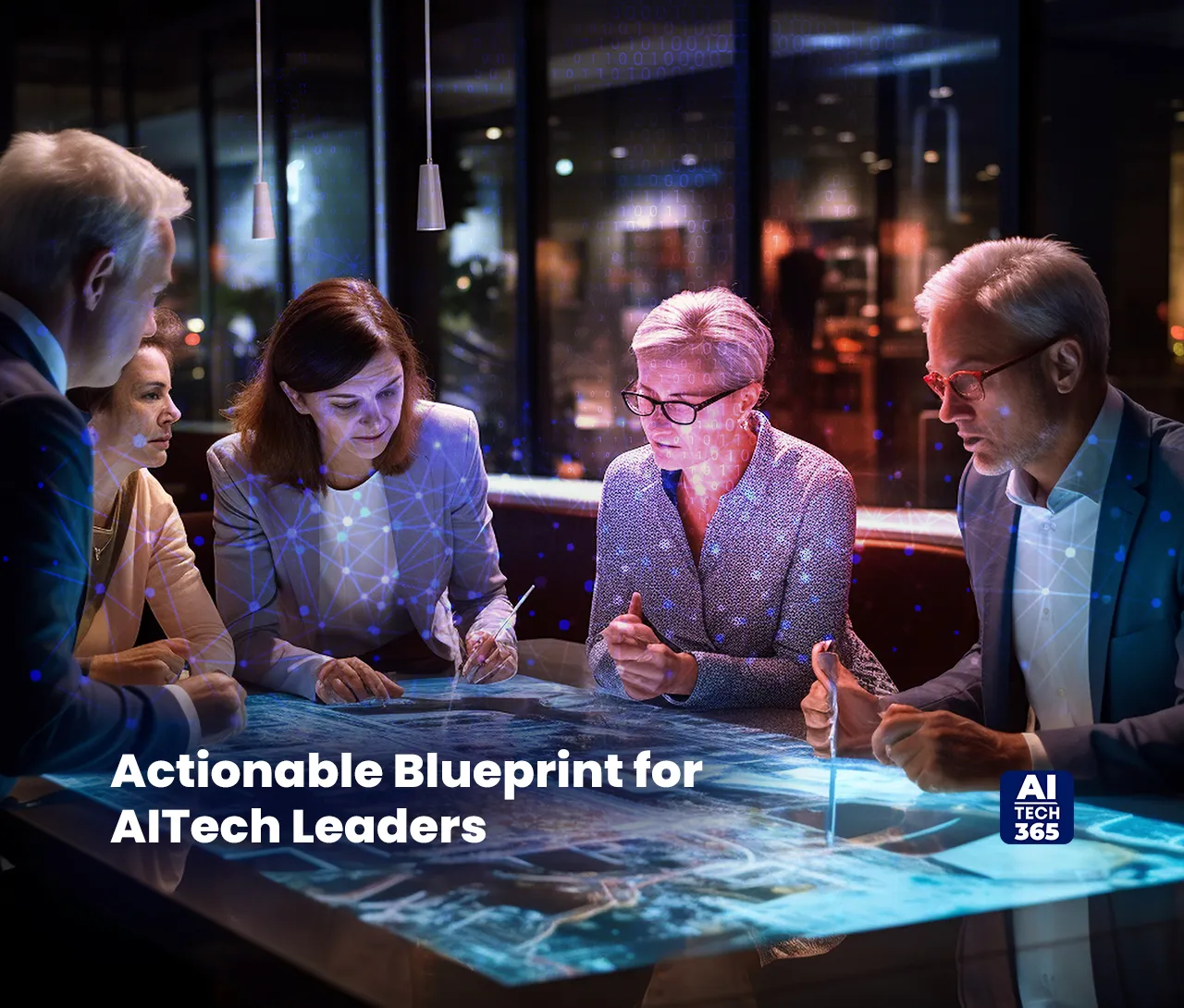
Start with Hybrid Intelligence: Pair AI with human SMEs in feedback loops. Cisco’s Crosswork Network Automation uses AI to suggest fixes but requires engineer approval, gradually increasing automation as trust builds.
Pressure-Test Your Data Diet: Audit data sources for diversity. Include external feeds—threat intelligence, supply chain statuses, even employee travel schedules affecting on-site repairs.
Measure What Matters: Track metrics like ‘Mean Time to Prediction’ (MTTP) and ‘False Positive Fatigue Rate.’ A model that complains too often gets ignored.
Ethical Guardrails: Ensure AI doesn’t disproportionately deprioritize low-revenue regions. Transparency in decision-making is critical for regulatory compliance.
Conclusion
As AI matures from prediction to prescience, network analytics is shedding its reputation as a cost center. It’s becoming a strategic differentiator—the difference between being the retailer that withstands Black Friday traffic surges and the one making headlines for all the wrong reasons. For AITech leaders, the mandate is clear: Treat your network not as cables and servers, but something as important as a person. Nurture it with AI’s observational prowess, and it will reward you with something priceless—anticipation.
In this new paradigm, the most successful enterprises won’t be those who fix problems fastest, but those whose problems never manifest at all. The future of network resilience isn’t just automated; it’s clairvoyant. And for pioneers willing to embrace AI’s predictive potential, that future is already here.