ChatGPT marked the beginning of AI hail. This trend continues to thrive even today as more people favor AI to increase their efficiency.
Now, the pressing question is: What’s next?
The answer is multimodal AI models. Within the ongoing AI revolution, this is one of the most promising trends. Multimodal generative AI models can combine different kinds of data to produce an output that might contain different kinds of results as well.
In this article, let’s learn the exciting possibilities that lie ahead with multimodal generative AI.
What is Multimodal AI?
Multimodal AI represents a cutting-edge advancement in artificial intelligence, integrating diverse data types like text, images, audio, and video to refine machine learning and decision-making processes. Unlike conventional single-modal AI, which focuses on one data type, multimodal harnesses the strengths of various modalities to deliver more accurate insights, informed conclusions, and precise predictions for real-world challenges.
Through training and leveraging diverse data types, multimodal artificial intelligence systems exhibit superior performance across a spectrum of applications. These applications span from video generation and character creation in gaming to content translation and the development of customer service chatbots, among others.
One example of multimodal AI is Google’s groundbreaking multimodal model, Gemini. This innovative system possesses the remarkable ability to process inputs from different modalities interchangeably. For instance, when presented with a photo depicting a plate of cookies, Gemini can discern the visual cues and generate a corresponding written recipe as output. Conversely, it can also interpret textual descriptions of recipes and generate visual representations, such as images or videos, providing a comprehensive understanding across modalities.
What is the Difference Between Single Modal And Multimodal AI?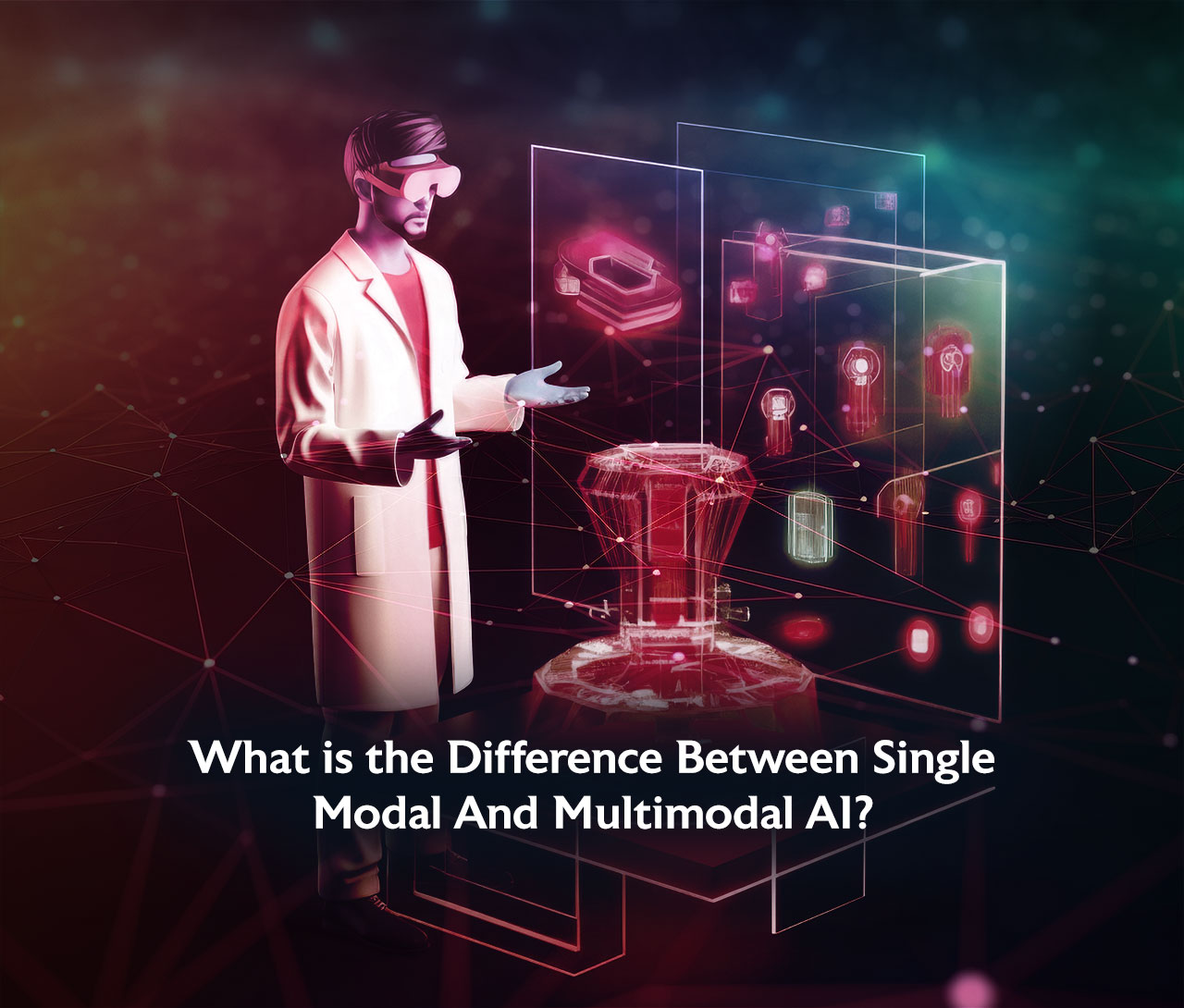
Single modal AI is designed to work with a single source or type of data, tailored to a specific task. This approach involves using a separate neural network for each type of data, such as financial data or image data, and analyzing it independently. In contrast, multimodal artificial intelligence processes data from multiple sources, such as video, images, speech, sound, and text, allowing for a more comprehensive and nuanced understanding of the environment or situation. Multimodal artificial intelligence utilizes multiple neural networks, each responsible for processing a specific modality, and combines the relevant information from different modalities using a fusion module. This integration of diverse data modalities leads to more accurate and informative outputs, enabling multimodal artificial intelligence systems to understand context, recognize patterns, and establish connections between different inputs.
Applications of Multimodal AI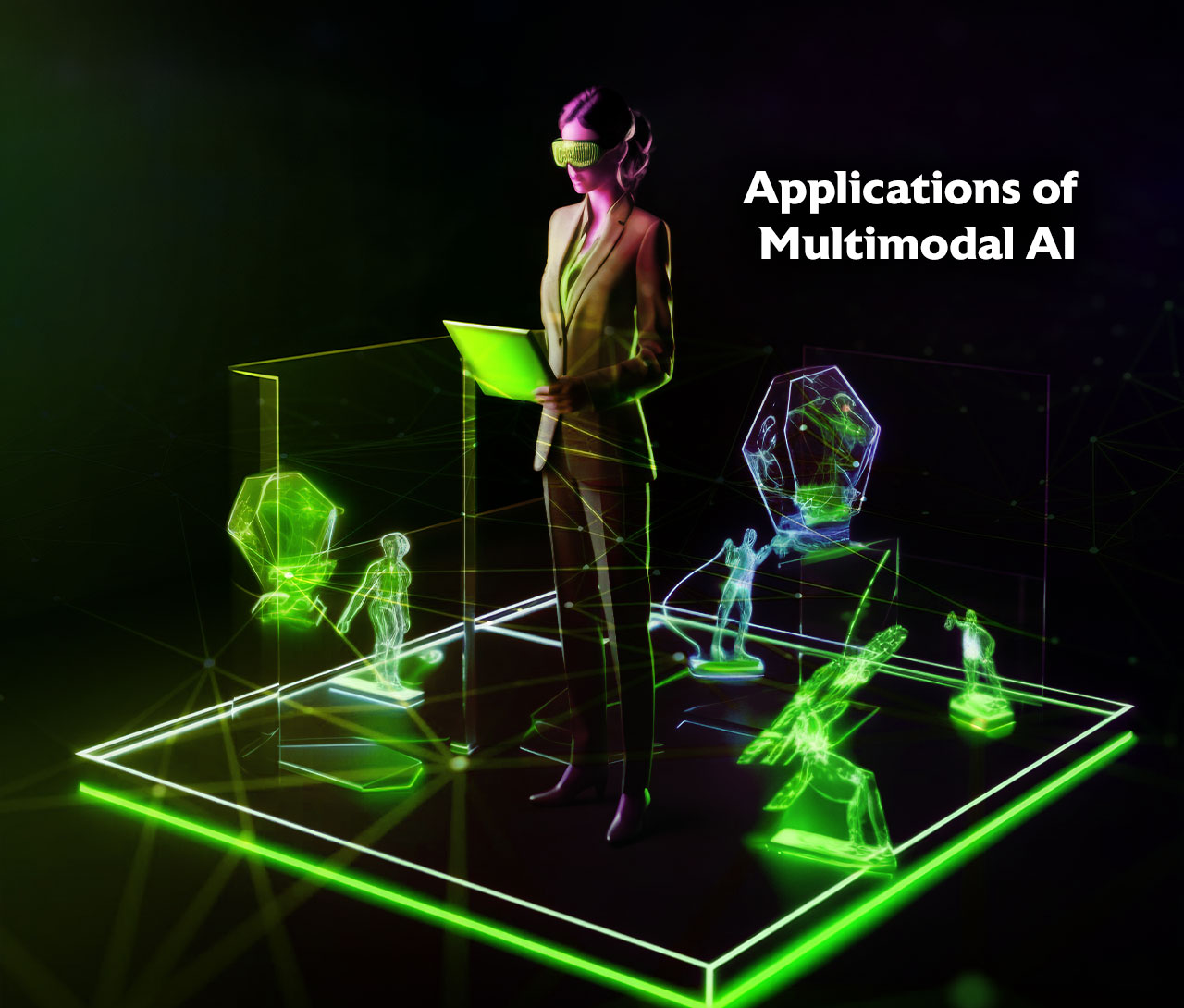
Multimodal learning empowers machines with the ability to acquire new “senses,” thereby enhancing their accuracy and interpretative capabilities. This advancement is ushering in a multitude of new applications across sectors and industries, including:
Augmented Generative AI:
The emergence of multimodal models such as Gemini, GPT-4 Turbo, or DALL-E marks a notable advancement in generative AI. These models introduce unparalleled capabilities, enriching user interactions both in input and output. Whether handling prompts across various modalities or creating content in multiple formats, the potential of multimodal artificial intelligence agents seems boundless.
Autonomous Cars:
Multimodal artificial intelligence plays a pivotal role in the advancement of self-driving cars. These vehicles utilize a variety of sensors to collect data from their environment in diverse formats. Multimodal learning is indispensable for enabling these vehicles to integrate and process these diverse sources efficiently, making intelligent decisions in real time.
Biomedicine:
The burgeoning availability of biomedical data from sources such as biobanks, electronic health records, clinical imaging, medical sensors, and genomic data is driving the creation of multimodal AI models in the field of medicine. These models can process data from multiple modalities to unravel the complexities of human health and disease, as well as aid in making informed clinical decisions.
Earth Science and Climate Change:
The proliferation of ground sensors, drones, satellite data, and other measurement techniques is expanding our understanding of the planet. Multimodal artificial intelligence plays a pivotal role in accurately integrating this diverse information and developing new applications and tools. These tools can assist in tasks such as monitoring greenhouse gas emissions, forecasting extreme climate events, and facilitating precision agriculture.
What is Unimodal Vs Multimodal AI?
Unimodal AI refers to systems that work with a single source or type of data, utilizing separate neural networks for each data type. In contrast, multimodal artificial intelligence processes data from multiple modalities, combining and aligning information to achieve a more comprehensive understanding.
By utilizing multiple neural networks and fusion modules, multimodal artificial intelligence systems can simulate human perception, leading to improved decision-making and accurate predictions for complex problems. Multimodal uses various data modalities, enabling a more holistic and nuanced approach to artificial intelligence.
Future of Multimodal AI
Multimodal AI represents a significant leap forward in the ongoing evolution of generative AI. The swift progress in multimodal learning is driving the emergence of novel models and applications tailored to diverse objectives. Yet, we are merely scratching the surface of this transformative journey. As advancements continue to merge additional modalities and refine techniques, multimodal artificial intelligence is poised to expand even further.
Nonetheless, alongside its immense potential, multimodal generative AI brings forth substantial responsibilities and complexities that demand careful consideration. Addressing these challenges is crucial to foster an equitable and enduring future.