Natural Language Processing (NLP) in healthcare offers exciting chances. It helps sort through lots of new information and uses it to improve results, save money, and give the best care possible. This blog discusses NLP use cases in healthcare and how it improves human lives. Let’s dive in.
What is NLP?
Natural language processing (NLP) is a specialized field of artificial intelligence (AI) that enables computers to comprehend and interpret human language.
Here’s how it operates: NLP systems start by pre-processing data, which involves cleaning up the dataset to make it more organized. This may include breaking down text into smaller units called “tokens” through a process known as tokenization. Pre-processing simplifies the dataset, making it easier for the NLP system to understand.
Following pre-processing, the system applies algorithms to the text for interpretation. The main algorithms used in NLP are rule-based systems, which rely on predefined grammatical rules to interpret text, and machine learning models, which utilize statistical techniques and improve over time through exposure to training data.
What is the use of NLP in Pharma?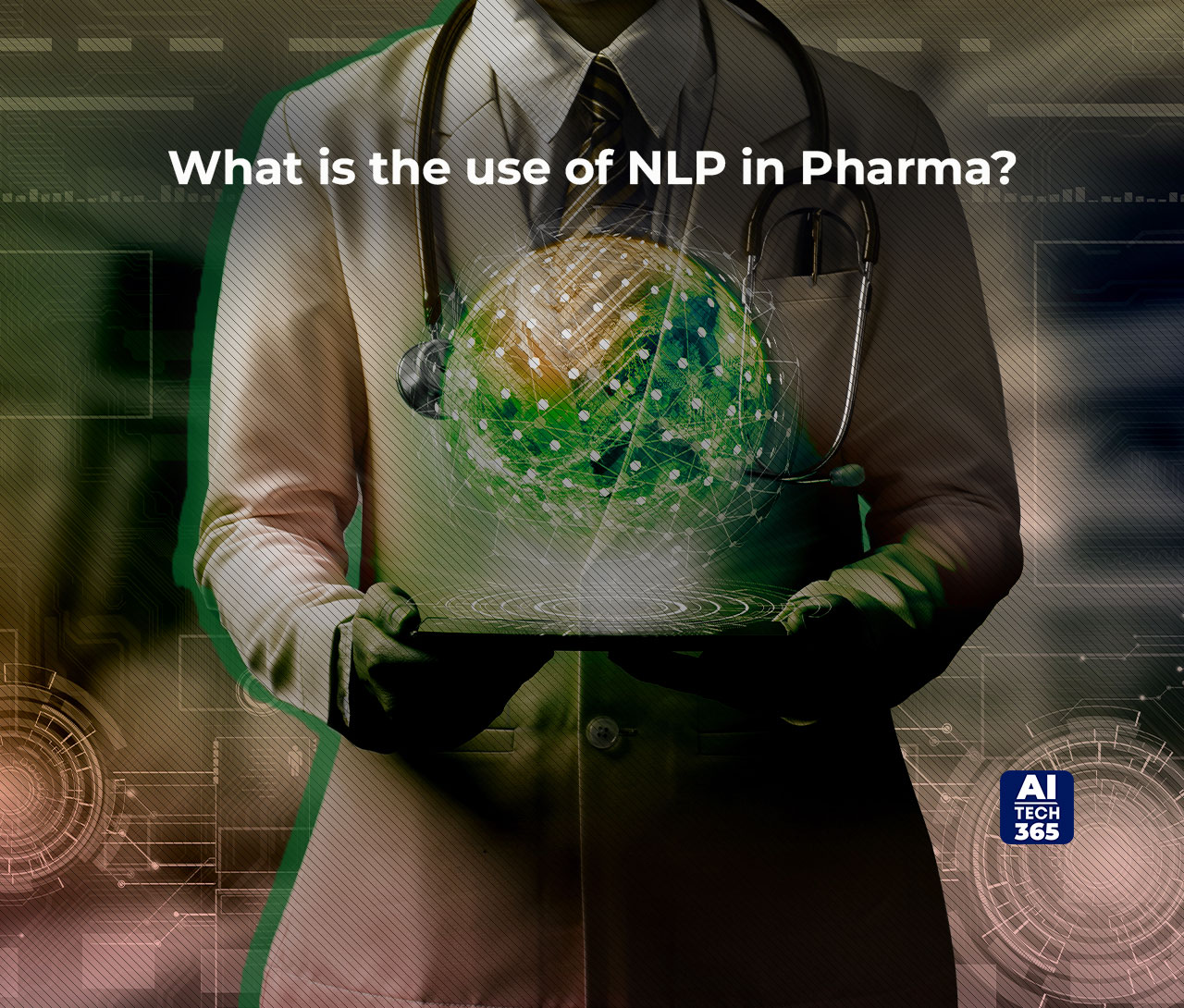
The NLP use cases in the healthcare industry highlight its significance across various applications.
- Data Analysis and Insights: NLP expedites big data analysis in the pharmaceutical sector, offering more precise results swiftly. It aids in comprehending customer feedback, improving medical care, and identifying new diseases.
- Drug Discovery and Research: In drug discovery, NLP mines electronic health record (EHR) data, extracting insights for commercial benefits. It swiftly identifies opportunities from diverse sources, enabling better care and efficacy.
- Text Mining and Decision Support: NLP text mining extracts crucial data from unstructured text, aiding decision-making from molecule to market. It facilitates gene-disease mapping, target selection, biomarker discovery, and safety analysis.
- Data Management and Analysis: Pharmaceutical companies use NLP to convert unstructured text into structured data for effective analysis. It transforms data analysis, enhancing drug development processes.
- Customer Feedback and Treatment Improvement: NLP enhances understanding of customer needs, facilitating the delivery of optimal treatments through ongoing care and new drug development. It improves drug efficacy by analyzing patient-reported outcomes.
- Drug Safety and Development: NLP automates text mining, enabling pharmaceutical firms to extract valuable insights from vast unstructured data. It enhances drug safety by analyzing extensive text-based data.
- Efficient Data Collection and Patent Analytics: NLP accelerates data processing and standardizes vast amounts of unstructured data into actionable insights swiftly. It simplifies patent literature mining for assessing patentability and freedom to operate.
How NLP can be used in healthcare?
Natural language processing is revolutionizing the healthcare field by providing predictive analysis tools for identifying health disparities across various demographic segments. It revolutionizes patient outcomes and the way healthcare is delivered by turning unstructured medical records into insightful knowledge.
NLP expedites big data analysis and report production for providers and supports disease diagnosis, treatment planning, and clinical research through topic modeling and semantic analysis. NLP also helps to improve clinical documentation, which increases physician productivity and decreases time spent on electronic health records.
Moreover, it is used in predictive analytics in the healthcare industry to help solve population health problems by identifying people who are at risk and facilitating more efficient surveillance.
3 Best NLP Use Cases in Healthcare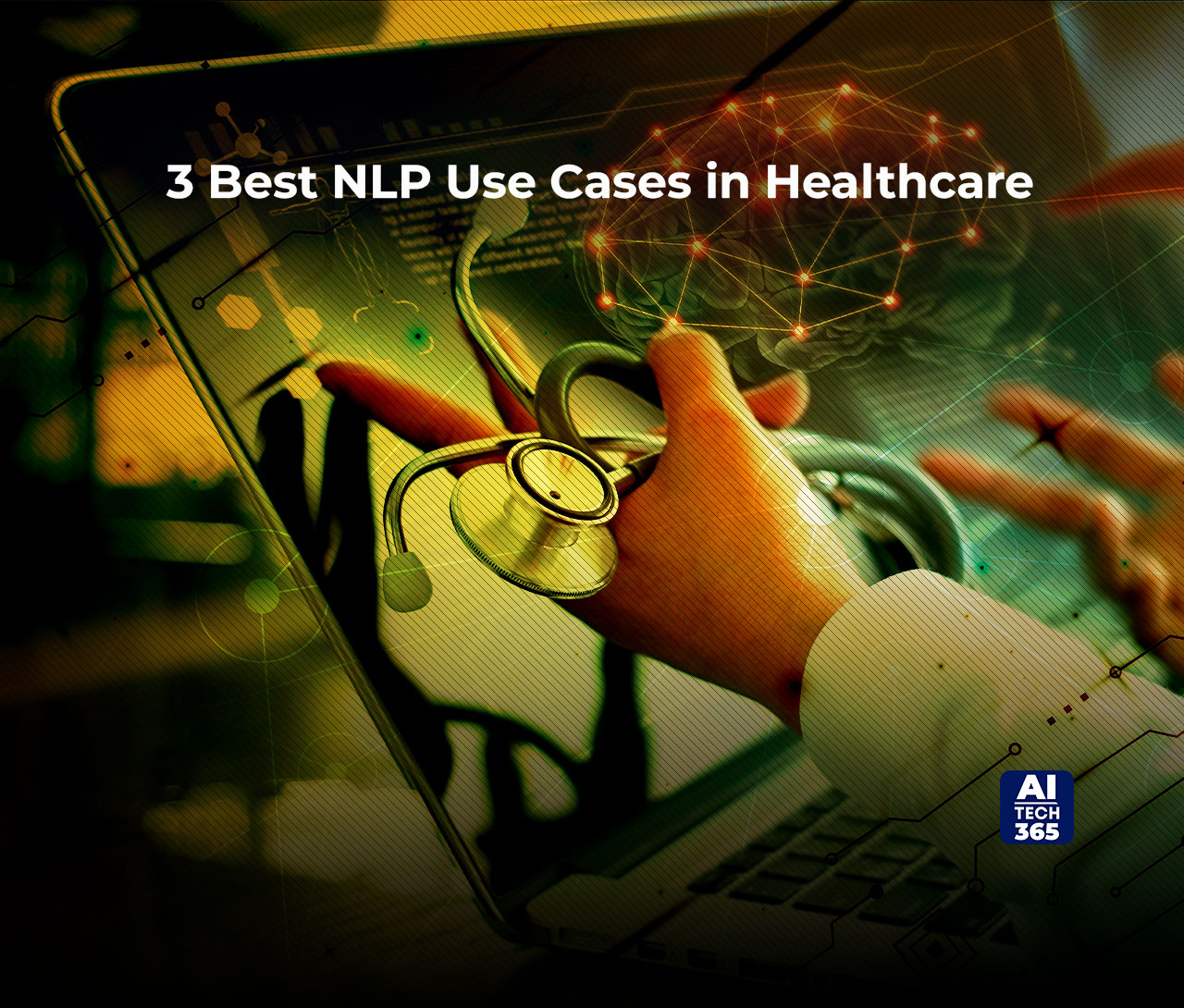
Below are the top three NLP use cases in healthcare that everyone should know about.
Documentation
The use of NLP in clinical notes (documentation) streamlines the process for clinicians, freeing them from cumbersome EHR systems and allowing more focus on patient care. Both speech-to-text dictation and structured data entry methods have proven invaluable. Technologies like Nuance and M*Modal utilize team-based speech recognition and standardized vocabularies to capture structured data efficiently at the point of care.
These NLP technologies extract pertinent information from speech recognition tools, significantly enhancing the analytical data used for Value-Based Care (VBC) and Population Health Management (PHM) initiatives. This leads to improved outcomes for clinicians. Looking ahead, NLP tools are likely to extend to analyzing public datasets and social media to identify Social Determinants of Health (SDOH) and assess the effectiveness of wellness-oriented policies.
Speech Recognition
NLP use cases in healthcare, specifically in speech recognition enables clinicians to transcribe notes for efficient EHR data entry. Front-end speech recognition frees physicians from dictation tasks, allowing them to remain at the point of care. Meanwhile, back-end technology detects and rectifies transcription errors before human proofing.
As the speech recognition market expands, it’s anticipated to reach a projected size of $8.53 billion by 2024. Despite the market for speech recognition technologies nearing saturation, certain startups are innovating within the space. They are leveraging deep learning algorithms for mining applications, thus unlocking a realm of broader possibilities.
Redacting Sensitive Data from Clinical Trial Documents
Natural language processing is a powerful tool for managing large volumes of documents and unstructured data. However, it also plays a crucial role in redacting sensitive information from clinical trial documents. Various data anonymization tools are available to assist in identifying and removing patients’ details from clinical trial files and databases. These records typically contain confidential information about patient health and treatment regimens.
Compliance with regulations such as HIPAA and the European Medicines Agency (EMA) is essential, as mishandling sensitive medical data can result in regulatory penalties or legal consequences. Thus, the redaction of medical records is paramount. NLP offers an efficient solution for removing such sensitive information, thereby safeguarding patients against risks such as identity theft. This is easily one of the best NLP use cases in healthcare.
End Note on NLP Use Cases in Healthcare
Without any doubt, NLP use cases in healthcare offer a multitude of advantages, extending beyond clinical notes analysis. By deploying NLP solutions, healthcare organizations can harness a variety of algorithms to identify and predict specific situations among patients, ultimately enhancing care delivery and streamlining workflows. Although the industry is working to improve its data capacities for the effective deployment of NLP tools, the potential for NLP and other machine learning (ML) tools to oversee clinical decision support and patient health explanations is monumental. As a result, NLP and ML are poised to play a pivotal role in revolutionizing healthcare by providing advanced clinical decision support and improving patient health management.