In the fast-paced world of enterprise tech, one trend stands out: the rise of large quantitative models (LQMs). By 2025, advanced computational systems will be essential for B2B companies. They will shape competitiveness, boost operational efficiency, and enhance strategic foresight. Tech leaders need to grasp how to use LQMs. This skill will set apart the innovators from those who struggle to keep up.
The Evolution of Decision-Making Which is From Intuition to Algorithmic Precision
For decades, B2B companies used human skills and old-school analytics to make decisions. These methods worked, but they often struggled with the complexity of today’s markets. Large quantitative models are advanced tools. They handle huge datasets, find hidden patterns, and provide useful insights. These processes happen at speeds we could not have imagined a few years ago.
LQMs are different from traditional models. They combine various data streams into one analytical engine. This includes market trends, supply chain variables, customer behavior, and geopolitical factors. Consider a global manufacturer optimizing its supply chain. Traditional tools might analyze historical demand or supplier performance in isolation. An LQM can show disruptions in real time. This includes trade wars, climate events, and material shortages. It offers dynamic recommendations to help reduce risk.
LQMs mark a shift from reactive to predictive business strategies. Early adopters in logistics, energy, and finance gain a lot. They enjoy better cost efficiency and more accurate decision-making. Many organizations are still hesitant. This is often because of misconceptions about complexity or resource needs. A common myth is that LQMs need petabytes of data to work well. Mid-sized companies can use these models. They should focus on high-quality, domain-specific datasets. It’s better than just chasing large amounts of data.
Why Large Quantitative Models Will Dominate 2025
The value proposition of LQMs hinges on scalability, adaptability, and contextual intelligence. Scalability lets these models manage rapid data growth. IoT devices, edge computing, and 5G networks produce a lot of data. This is important. Adaptability lets LQMs grow with market changes. They learn from new data without needing manual adjustments. Contextual intelligence is a key feature. It helps models understand unstructured data like emails, social sentiment, and regulatory documents. Then, they can use this information in decision-making processes.
Take the example of a multinational bank assessing credit risk. Legacy systems might evaluate loan applications based on credit scores and financial histories. An LQM can look at cash flow trends, economic signs, and news about the borrower’s industry. This helps predict default risks more accurately. This depth of analysis isn’t just incremental; it’s revolutionary.
Regulatory pressures are also accelerating adoption. Compliance rules in healthcare and finance now require clear and trackable decision-making. LQMs can track outcomes to specific data inputs. This helps meet the needs of regulators and stakeholders. A pharmaceutical company can use LQMs to make drug trials smoother. This helps regulators see how patient details, genetic info, and trial setups affect results. This way, they can meet strict transparency laws.
Overcoming Implementation Challenges
Despite their potential, integrating LQMs into enterprise workflows isn’t without hurdles. Data quality remains a persistent issue. In 2025, 64% of organizations identified data quality as their top data integrity challenge, up from 50% in 2023. Models trained on incomplete or biased datasets will produce flawed recommendations. A European retailer faced a tough lesson. An LQM aimed to boost inventory levels. However, they mixed up seasonal sales spikes with long-term demand trends. This mistake caused overstocking and wasted capital. To avoid problems, companies need to focus on data hygiene. This means cleaning, labeling, and validating datasets before using them in models.
Computational costs also pose a barrier. Training and keeping LQMs needs big infrastructure investments. This is especially true for groups without in-house expertise. Cloud-based solutions and partnerships with specialized AI firms help with this challenge. However, tech leaders still need to consider upfront costs versus long-term ROI. A practical approach is to start with modular implementations. A logistics company might start by using an LQM to improve route planning. Then, it could expand its use to demand forecasting or warehouse automation.
Ethical considerations add another layer of complexity. LQMs affect hiring, pricing, and resource use. So, companies must protect themselves from algorithmic bias. A North American healthcare provider faced criticism. Its patient prioritization model unintentionally favored groups with better access to care. Fixing these issues requires careful auditing and a variety of training data. Tech leaders must not ignore this responsibility. To tackle these challenges, organizations can take proactive steps. They might set up ethics committees or work with third-party auditors. They also build trust with stakeholders.
Actionable Strategies for Tech Leaders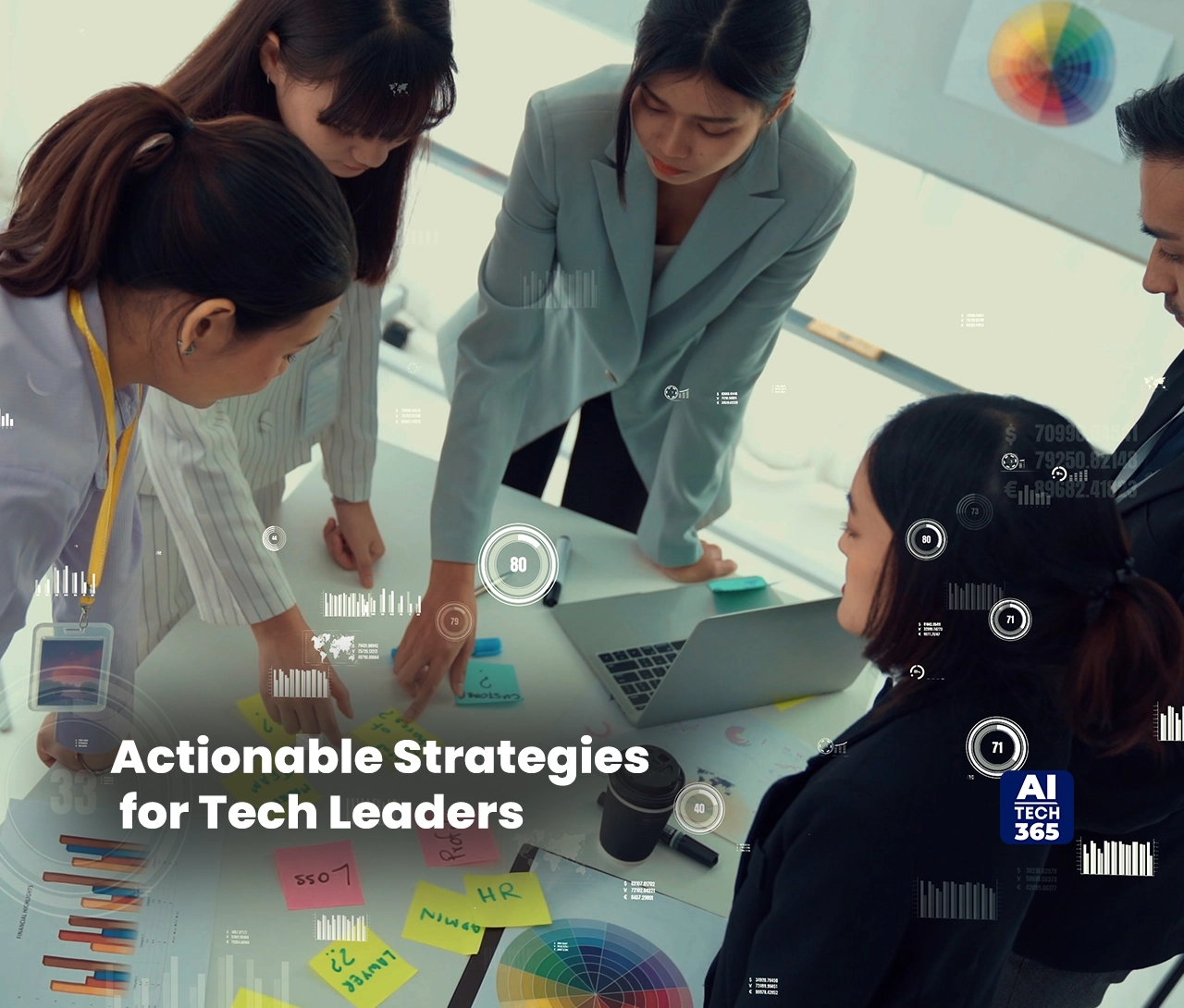
For enterprises ready to embrace LQMs, success starts with alignment. Models should match clear business goals. This includes cutting waste, personalizing B2B experiences, and predicting market changes. Data scientists must work with domain experts. Without their knowledge, even the best models can turn into mere academic exercises. A good example is a manufacturing firm. They paired data engineers with supply chain experts. Together, they made an LQM that predicts equipment failures. The model used tips from experienced technicians. It looked at machine vibrations and maintenance history. This cut downtime by almost forty percent.
Investing in data infrastructure is equally critical. Siloed datasets inhibit LQMs’ ability to deliver holistic insights. Centralized data lakes and solid governance ensure models receive clean, relevant information. A mid-sized aerospace company cut production delays. They combined supplier performance data, maintenance logs, and weather patterns into one LQM-driven platform. The outcome was a clear view of operational risks. This allowed for proactive changes to production schedules and supplier contracts.
Finally, pilot programs are essential for building organizational buy-in. Focusing on a specific use case, like optimizing ad spend for one product line, helps teams show value. This approach keeps stakeholders from feeling overwhelmed. As confidence grows, enterprises can scale implementations across departments. A consumer goods company tried this by using an LQM to predict regional demand for a new product. The company cut excess inventory by twenty percent. Then, it expanded the model to its whole portfolio. This move boosted profit margins by making production and distribution more efficient.
The Future of LQMs
In the future, LQMs and new tech like quantum computing and neuromorphic hardware will show us new possibilities. Quantum-enhanced models can solve optimization problems in minutes. Right now, these problems take weeks to solve. This could change fields like pharmaceuticals and materials science. A quantum-powered LQM can quickly simulate how molecules interact. This speed is much faster than classical computers. As a result, it can speed up the development of life-saving drugs.
Neuromorphic systems, which take cues from the human brain, may help LQMs process sensory data right away. This advancement may lead to new uses in autonomous logistics and industrial IoT. Picture a warehouse where LQMs work with neuromorphic sensors. They change the paths of robotic pickers in real time. This happens based on shifts in inventory levels, package weights, and worker movements. Such systems could reduce errors, improve safety, and slash operational costs.
Another trend to watch is the democratization of LQM tools. Platforms like AWS SageMaker and Google Vertex AI let smaller companies use tools that big tech once kept to themselves. This change could create a fairer environment. It would spark innovation in fields like agriculture and renewable energy. A startup in agritech might use ready-made LQM tools. These tools help analyze soil health, weather patterns, and crop prices. This analysis helps farmers optimize planting schedules and boost yields.
Preparing for a Data-Driven Future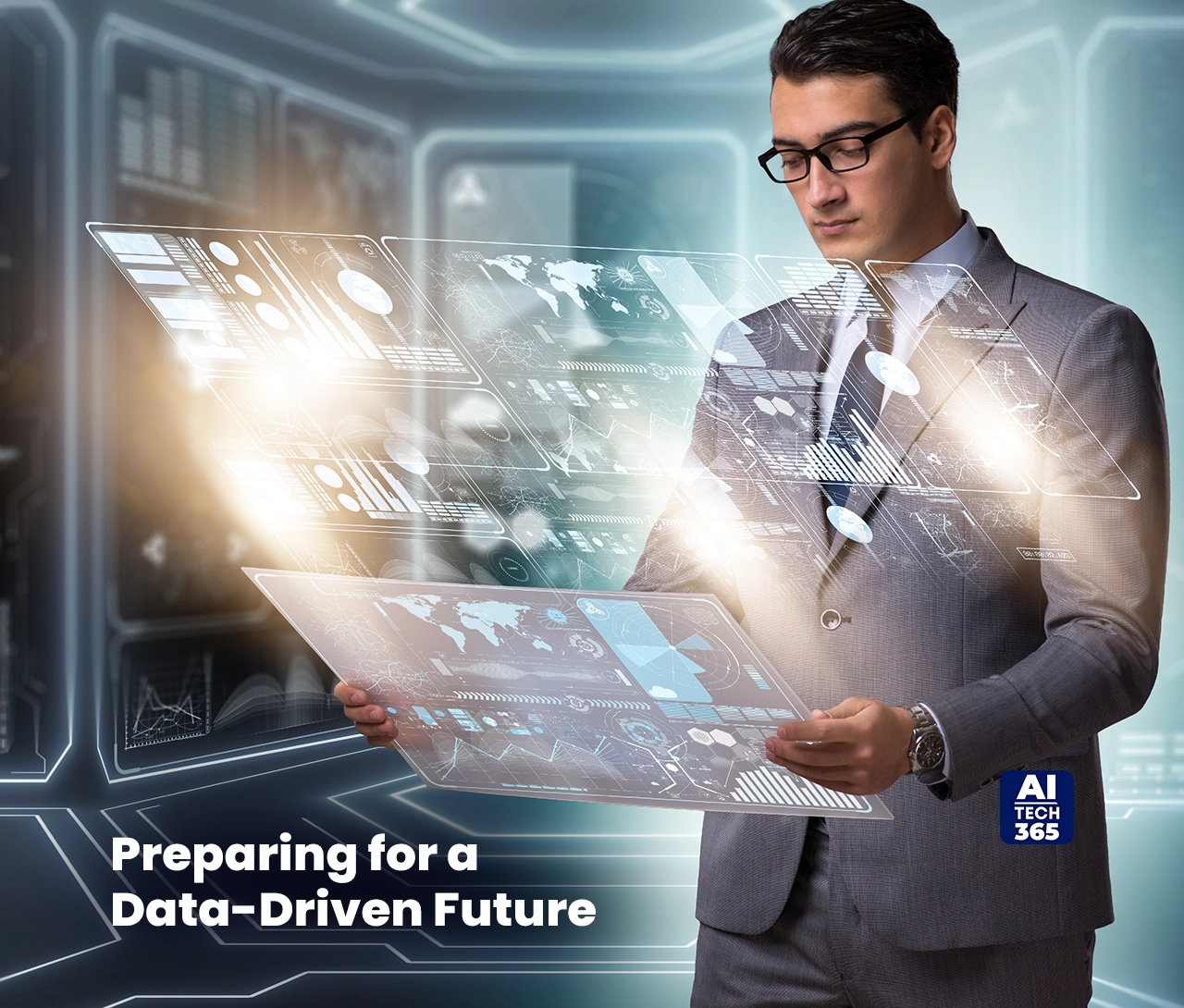
Tech leaders should note this: large quantitative models aren’t a far-off dream. They’re the core of next-gen enterprise strategy. Organizations that wait to adopt LQMs may fall behind. Competitors using LQMs can find trends, boost operations, and deliver great customer value.
Yet, technology alone isn’t the answer. A data-savvy workforce will be key for success in 2025. Also, promoting ethical AI use matters. Staying agile during changes is important too. Training programs that bridge the gap between technical and non-technical teams are essential. A financial services firm started workshops. In these sessions, data scientists explain LQM outputs to executives in simple terms. This helps collaboration and keeps everyone aligned with the strategy.
The era of intuition-driven leadership is ending. A new way of thinking is here. Now, algorithms guide decisions that match the challenges they address. For those willing to invest, experiment, and adapt, the rewards will be transformative. The question isn’t if your enterprise needs big models. It’s about how fast you can make them part of your strategy. The clock is ticking.
Final Thoughts
By 2025, using large quantitative models in B2B operations won’t just be a luxury; it will be a must. Early adopters of these tools will improve efficiency, spark innovation, and enhance customer satisfaction. Success needs more than using technology. It requires a cultural change. This means using data to make decisions, learning all the time, and being responsible. Tech leaders can help their organizations thrive by facing challenges directly. They should encourage teamwork in different areas and stay updated on new technologies. This way, they can succeed in the age of algorithmic precision. The future belongs to those who dare to reimagine what’s possible; and act with urgency.