In the progressively developing landscape of technology, the requirement for structured and intelligent systems has reached unmatched heights. Traditional computing designs, while transformative in their own right, are now struggling with respect to energy efficiency, flexibility, and real-time processing. Enter neuromorphic computing—a revolutionary innovation designed to copy the structure and functions of the human brain.
Neuromorphic computing is the convergence of neuroscience and computer engineering, offering a progressive take on processing of data and problem-solving. This article is your ultimate roadmap to neuromorphic computing. We are going to delve into its foundational principles, groundbreaking applications, daunting challenges, and future possibilities. Whether a tech enthusiast, an AI expert, or just curious about the evolution of computing, get ready for a wealth of valuable insights.
What is Neuromorphic Computing?
At its very heart, neuromorphic computing is an architectural example inspired by the neural networks of a human brain. As opposed to binary logic and sequential processing, neuromorphic systems may replicate the power of the human brain in terms of information processing by interlinked neurons and synapses. In using spiking neural networks (SNNs) and asynchronous event-driven architectures, the system can handle data in an extremely well-organized and parallelized fashion
Key Characteristics:
- Energy Efficiency: Neuromorphic chips use considerably less than standard CPUs and GPUs. This makes them well suited for low-energy applications.
- Parallelism: These systems have the ability to handle multiple computations at the same time, imitating the brain’s capability to process naturally.
- Adaptability: Neuromorphic systems can learn from new information and adapt to it. This helps in real-time decision-making and trouble-shooting.
For example, the human brain consumes approximately 20 watts of power when using roughly 86 billion neurons. Neuromorphic designs strive to recreate the same level of planning, a feat which cannot be attained through conventional methods of computing.
Why Neuromorphic Computing Matters?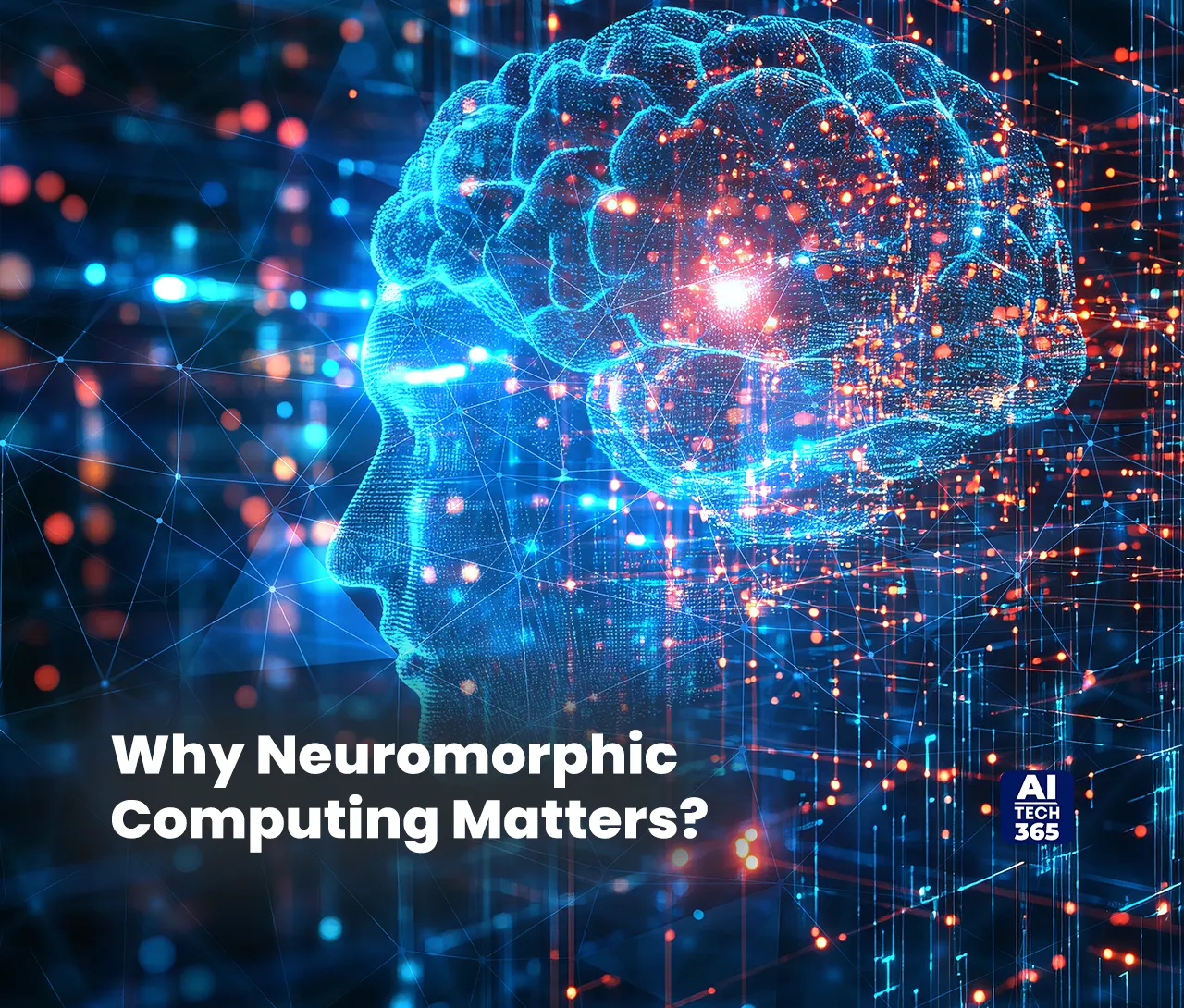
As industries become progressively data-driven, the setbacks of traditional computing architectures are becoming evident. Neuromorphic computing resolves these challenges by offering:
-
Enhanced Energy Efficiency:
Traditional supercomputers need huge amounts of energy to perform tasks such as AI model training. Neuromorphic chips, on the other hand, achieve identical outcomes with a small part of the energy.
-
Real-Time Processing:
Applications like robotics, autonomous vehicles, and healthcare need real-time data analysis and decision-making. Neuromorphic systems are ideal in such cases, thanks to their parallel and event-oriented architectures.
-
Scalability and Miniaturization:
As devices become smaller and more unified, the compressed design and capability of neuromorphic chips make them ideal for edge computing and IoT devices.
Also Read: All You Need to Know About Human Augmentation
Applications of Neuromorphic Computing
The flexibility and adaptability of neuromorphic computing has given access to a vast range of applications across several industries. Let us dive deeper into its revolutionary capabilities.
-
Healthcare
Neuromorphic computing is expected to transform the medical field. Some of its remarkable end uses are:
- Prosthetics: Advanced brain-machine interfaces of neuromorphic systems facilitate the control of prosthetic limbs, offering enhanced limb functions and user experience.
- Neurological Diagnostics: By examining neural signals, neuromorphic chips can discover early signs of neurological diseases like Alzheimer’s, epilepsy, and Parkinson’s disease.
- Wearable Devices: Low-power, real-time processability makes these chips ideal for use in wearable devices, such as smart watches and fitness trackers, to monitor health.
-
Autonomous Vehicles
Neuromorphic systems are also transforming the automotive industry. They can:
- Process sensory data from cameras, LiDAR, and radar in real time.
- Amplify decision-making speed and precision, securing safer autonomous driving.
- Minimize consumption of energy, increasing battery life in electric vehicles.
-
Robotics
Robots fuelled by neuromorphic chips feature:
- Lifelike Adaptability: Enhanced association with humans, allowing robots to respond to emotional signals and environmental shifts.
- Enhanced Efficiency: Real-time learning and adaptability in industrial applications, managing workflows and performance output.
-
Edge Computing and IoT
The power to process data locally, without depending on consolidated servers, makes neuromorphic systems well-preferred for edge computing and IoT. Applications include:
- Smart Homes: Devices that can manage and optimize energy use of the user preferences.
- Environmental Monitoring: Sensors skilled in real-time data analysis for climate and pollution monitoring.
- Wearable Technology: Portable devices featuring advanced tech with minimal consumption of power.
Data-Driven Neuromorphic Campaigns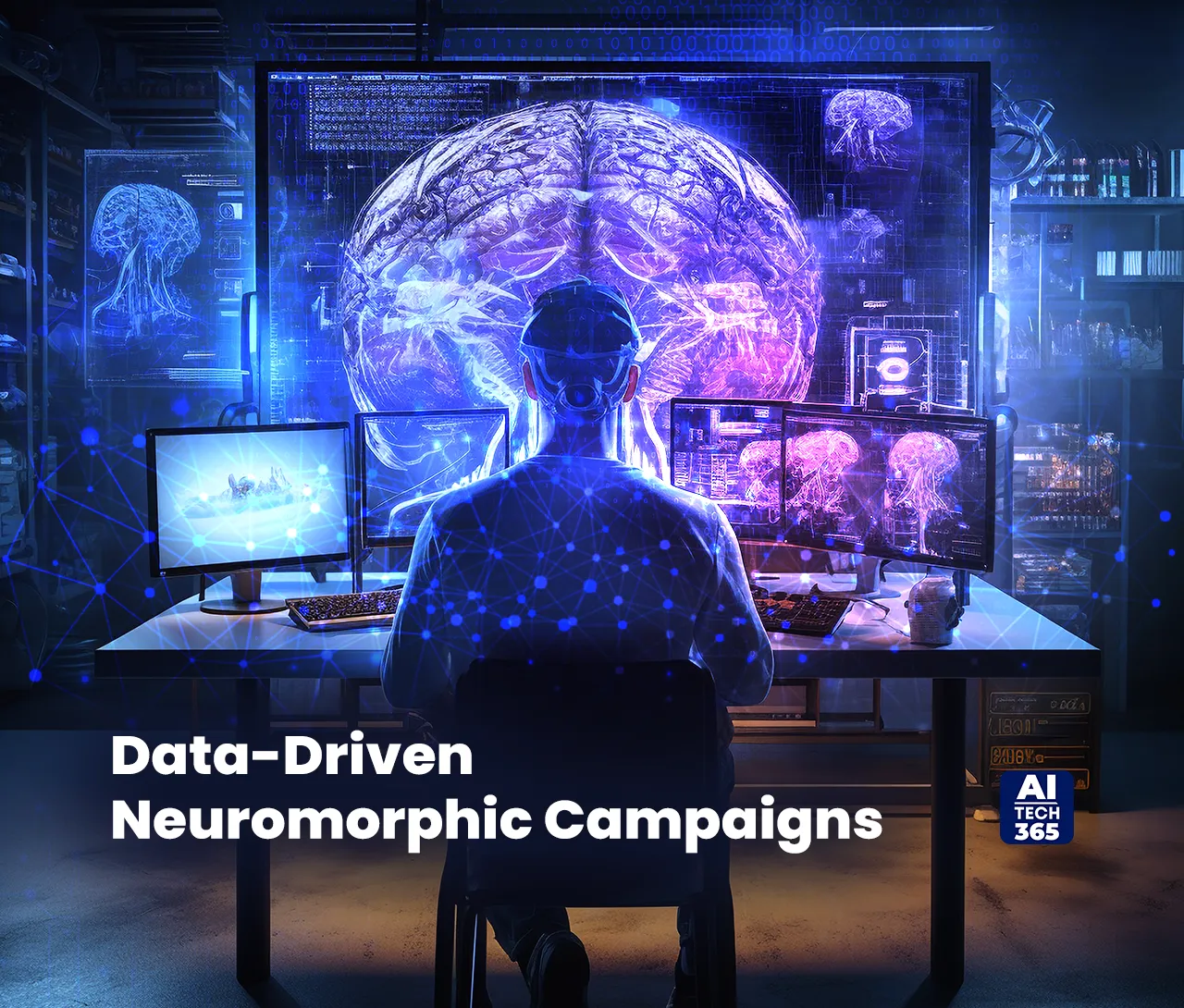
Leveraging Foot Traffic Analytics:
The most favorable placement for neuromorphic systems in IoT networks is largely determined by data collection procedures such as foot traffic analysis and sensor networks. This guarantees high-performance and relevance in both urban and commercial settings.
Integration with Omni channel Strategies:
Neuromorphic computing can flawlessly connect with wider multi-channel campaigns. For example, combining data from neuromorphic sensors with cloud-based analytics platforms can create homogenous and thoughtful experiences for customers.
Real-World Examples
-
IBM TrueNorth:
An next-gen chip showcasing 1 million neurons and 256 million synapses. TrueNorth is energy-efficient and can recognize patterns quickly. This chip consumes only 70 milliwatts of energy for its operations.
-
Intel Loihi:
Made for on-chip learning, Loihi is used in robotics and AI research to increase resilience and speed.
-
Brain Scales:
A European project, BrainScaleS offers quicker neural simulations, assisting in further innovations in neuromorphic research.
Challenges in Neuromorphic Computing
While the capabilities are enormous, neuromorphic computing does not come without challenges:
- Hardware Complexity: Designing neuromorphic chips with accurate neuron and synapse positioning still remains a technical hurdle.
- Software Development: Current programming structures are not aligned with neuromorphic architectures, and requires new tools and languages.
- Adoption and Awareness: Industries need to appreciate the value and invest to facilitate the transition to neuromorphic systems.
The Future of Neuromorphic Computing
The next decade promises noteworthy developments in neuromorphic computing:
- Widespread IoT Integration: From smart cities to customized wearables, neuromorphic systems will power a new era of intelligent devices.
- Breakthroughs in AI: Faster and more intuitive AI systems capable of human-like reasoning and decision-making.
- Medical Innovations: Transforming patient care with accurate diagnostics and real-time monitoring systems.
Conclusion
Neuromorphic computing represents a colossal jump in the advancement of technology. By replicating the brain’s unmatched efficiency and versatility, this model is set to redefine computing. From transforming healthcare to sanctioning smarter cities, the possibilities are endless.
As researchers and industries continue to come together, neuromorphic computing will not only push the boundaries of what machines can achieve but also inspire a future where technology operates effortlessly with the human experience. The journey toward brain-inspired machines has only just begun, and its potential is as vast as the human mind itself.